The Most Comprehensive Sharing for Video Dataset: Part 1, Action Recognition Datasets
In the current digital era, artificial intelligence has permeated various fields, becoming a pivotal force driving technological innovation and societal development. From early rule-based simple algorithms to the complex intelligent systems represented by deep learning today, every major breakthrough in artificial intelligence has been closely linked to the exponential growth of data and leaps in processing capabilities. Video data, as an information-dense and visually intuitive data source, plays a crucial role in this evolutionary process. With the massive generation and widespread application of video data, video understanding technology has become increasingly important. It enables automatic classification, annotation, and retrieval of video content, enhancing the efficiency and accuracy of video processing, and providing robust support for various applications such as video surveillance, intelligent transportation, film production, and online education.
Video datasets provide large models with rich spatiotemporal information, enabling them to learn dynamic features such as object motion, scene changes, and event progression. High-quality video datasets encompass a variety of scenes, actions, and contexts, which help improve the generalization capabilities of models, making them more robust when dealing with the complexities of the real world. In emerging tasks such as text-to-video generation, comprehensive and diverse video datasets are indispensable, as they provide the models with the knowledge to map from text to visual sequences.
Action Recognition Datasets: The Key to Unlocking the Understanding and the Generation of Video
Action Recognition aims to accurately classify and deeply understand human or object actions in videos. By mining the rich spatiotemporal information contained in video sequences, it automatically extracts key action features and efficiently matches these features with predefined action categories, thereby accurately determining the type of action occurring in the video.
As an important subset of video datasets, action recognition datasets focus on the task of identifying and classifying human actions. They contain a diverse range of human action video samples, covering everything from simple limb movements (such as walking, running, waving) to complex behavioral activities (such as various actions in sports competitions, operational processes in industrial production, and behavioral expressions in social interactions). Each action video is precisely annotated with action categories, and some datasets further provide details such as the start and end times of actions, detailed descriptions of actions, and the subjects and objects involved in performing the actions.
In the field of generative artificial intelligence, action recognition datasets play a crucial role:
- They provide a solid foundation and rich material for action generation. Generative AI models aim to create new, realistic content, and the vast number of action samples in action recognition datasets enable models to learn the detailed features and patterns of different actions. For example, in virtual reality (VR) and augmented reality (AR) applications, to create lifelike virtual character movements, models need to rely on action recognition datasets to learn the natural fluidity and coordination of human actions, thereby generating action sequences that conform to physical laws and human behavioral habits, greatly enhancing the realism and immersion of virtual scenes.
- They facilitate multimodal fusion. In practical applications, single-modal data often fails to meet the demands of complex tasks. By combining action recognition data with other modalities (such as images, audio, and text), models can achieve more comprehensive and in-depth information understanding and expression capabilities. For instance, in video content generation tasks, integrating text descriptions with action recognition data allows models to generate corresponding video actions based on given textual plots, enabling intelligent conversion from text to dynamic visuals. This provides new creative tools and efficient production methods for fields such as film production and advertising.
- They effectively enhance the generalization capabilities of models. Since the datasets include samples from various scenes, characters, and action types, models can be exposed to a wide range of action variations during training. This enables them to make reasonable inferences and creations when faced with new, unseen action generation tasks, reducing over-reliance on specific data and improving the adaptability and reliability of models across different application scenarios.
The existence of action recognition datasets opens up broad avenues for innovative applications of generative artificial intelligence in multiple fields. In film production, directors and special effects artists can use models trained on action recognition datasets to generate various fantastical and thrilling action effects, enriching the visual impact of movies. In game development, it enables the creation of more diverse, personalized, and naturally fluid character movements, enhancing the fun and immersive experience for players. In the field of intelligent robotics, robots can better understand human commands and intentions by learning human action patterns from action recognition datasets, achieving more precise and natural human-machine interactions. This expands the application scope of robots in scenarios such as home services, medical care, and industrial collaboration.
This article will introduce some classic action recognition datasets.
UCF-101
- Publisher: University of Central Florida
- Download Address: https://www.crcv.ucf.edu/research/data-sets/ucf101/
- Release Date: 2012
- Description: The UCF-101 dataset is a renowned video action recognition dataset, consisting of 13,320 video clips covering 101 different human action categories. The uniqueness of UCF-101 lies in its diverse action categories, encompassing a wide range of daily activities and sports. These 101 categories can be divided into five groups (body movements, human-human interactions, human-object interactions, playing musical instruments, and sports). Each video demonstrates a distinct action, ranging from gymnastics to sailing, fencing to weightlifting, and more.
The research team downloaded relevant videos from platforms such as YouTube to ensure the diversity and representativeness of the dataset. A series of selection criteria were applied during the process, such as limiting video duration, ensuring video clarity, and accurate labeling of action categories. During the annotation phase of the video clips, researchers conducted manual labeling and verification to ensure the precise correspondence between each video clip and its action label.
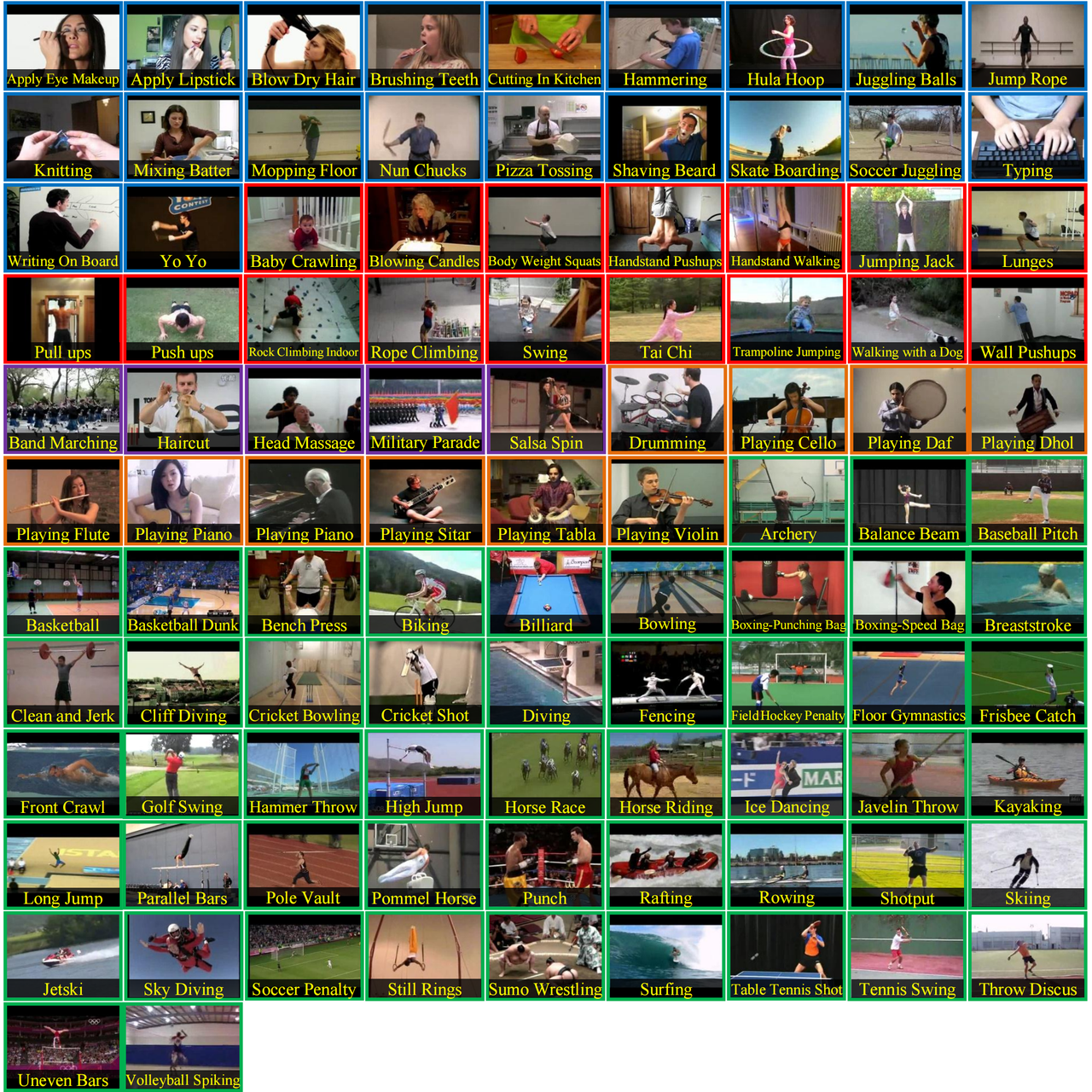
Kinetics
- Publisher: DeepMind
- Download Address: https://www.deepmind.com/open-source/kinetics
- Paper Address: https://arxiv.org/abs/1705.06950
- Release Date: 2017
- Description: Kinetics is a widely used large-scale video dataset designed to advance research in video action recognition and deep learning models. This dataset contains hundreds of thousands of video clips, covering 400 (Kinetics-400), 600 (Kinetics-600), and 700 (Kinetics-700) different human action categories, depending on the dataset version. The uniqueness of Kinetics lies in its large scale and high diversity of action categories, encompassing a wide range of complex human activities: each video demonstrates a specific action, ranging from daily activities to complex sports, social interactions to gameplay, and more.
The research team collected a vast number of videos from open platforms such as YouTube. Through a combination of automated and manual review steps, they ensured the coverage of diverse and representative action categories, ultimately refining the dataset to a specific number of visual tasks (e.g., 400, 600, or 700). During the video selection process, they applied a series of stringent filtering criteria to ensure video quality, action clarity, and label accuracy. Each video clip is accompanied by an action label, which is initially assigned through a semi-automated method and then manually verified to ensure high accuracy and consistency in annotations.
The Kinetics dataset, due to its large scale and detailed action categories, is widely used for training and evaluating various video understanding models, particularly in action recognition tasks. Although significant effort has been made to ensure annotation accuracy, the diversity and openness of its sources may still introduce some label noise. Nevertheless, this large-scale dataset provides researchers with ample resources to develop and validate advanced video analysis algorithms.
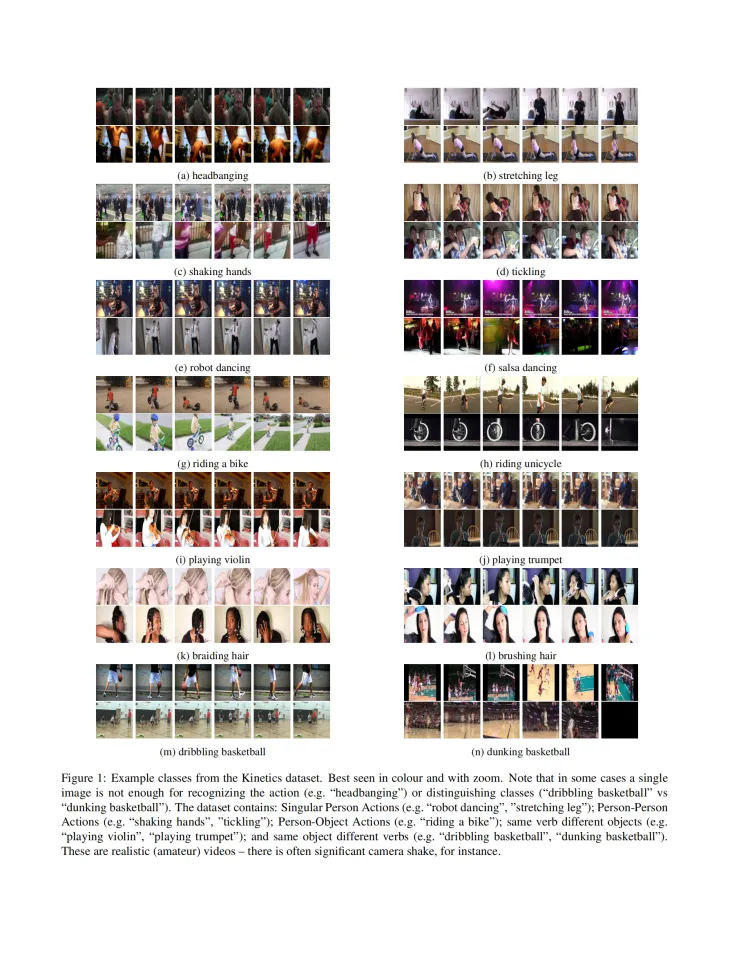
Charades
- Publisher: The Allen Institute for AI, Carnegie Mellon University
- Download Address: https://prior.allenai.org/projects/charades
- Paper Address: https://arxiv.org/abs/1604.01753
- Release Date: 2016
- Description: The Charades dataset consists of 9,848 annotated videos, with an average length of 30 seconds, showcasing activities performed by 267 individuals across three continents, totaling approximately 80 hours of footage. Each video is annotated with multiple free-text descriptions, action labels, action intervals, and categories of interacting objects. In total, Charades provides 27,847 video descriptions, 66,500 temporally localized intervals across 157 action classes, and 41,104 labels for 46 object categories.
The research team designed a novel method to collect and annotate this dataset. They first generated a long list of possible activities, which were then reenacted by users of Amazon Mechanical Turk. Participants recorded videos at home based on given descriptions. These descriptions were not simple, independent activities but rather complex, multi-step scenarios. For example, a video might depict a series of continuous actions, such as a person waking up, getting dressed, and having breakfast.
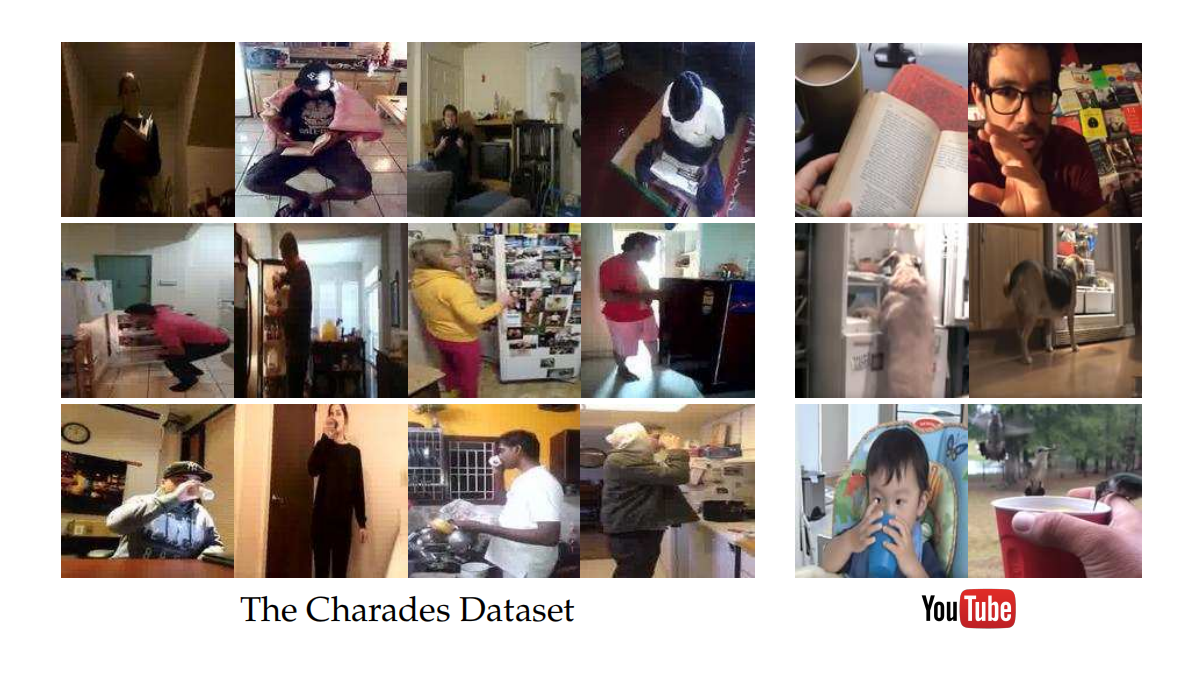
ActivityNet
- Download Address: http://activity-net.org/index.html
- Paper Address: https://ieeexplore.ieee.org/document/7298698
- Release Date: 2015
- Description: ActivityNet is a large-scale video dataset with a vast amount of data and a wide variety of activities. It contains up to 20,000 videos, totaling over 849 hours of footage, covering 200 different human activity categories. The distinguishing feature of ActivityNet lies in its extensive diversity of activities, which span daily life, sports, entertainment, and various other scenarios. Version 1.3 of the dataset includes a total of 19,994 untrimmed videos, divided into three disjoint subsets in a 2:1:1 ratio for training, validation, and testing. On average, each activity category contains 137 untrimmed videos. Each video has an average of 1.41 temporally annotated activities. The ground truth annotations for the test videos are not publicly available.
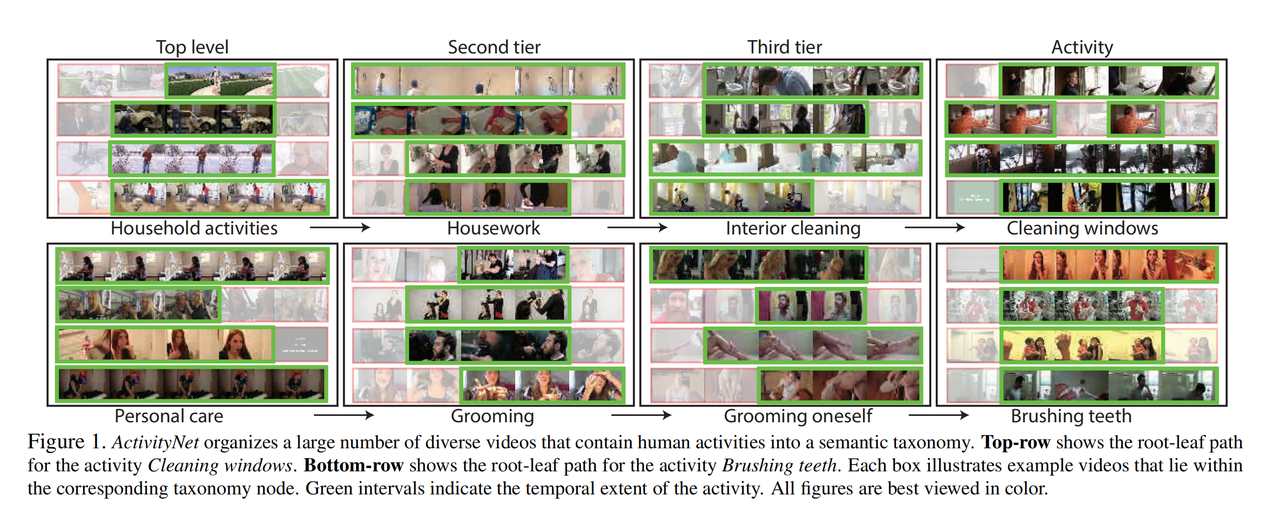
MMAct
- Download Address: https://mmact19.github.io/2019/
- Paper Address: https://ieeexplore.ieee.org/document/9009579
- Release Date: 2019
- Description: MMAct is a pioneering large-scale dataset for multi-person interaction actions, designed to advance research in video understanding and multimodal learning. This dataset includes various sensor data and video clips, covering a wide range of interpersonal interactions and independent action tasks. The uniqueness of MMAct lies in its focus on multimodal interaction content: each data segment showcases the actions and interactions of multiple individuals performing specific tasks, ranging from daily life activities to complex coordinated movements.
The research team generated a comprehensive list of activities by analyzing common interpersonal interaction scenarios. By filtering for "interaction tasks" in major categories, they focused on activities involving multiple senses and physical world interactions, ultimately refining the dataset to specific visual tasks. Subsequently, they designed a series of experiments to capture multimodal data, including video, depth data, and inertial measurement units (IMUs), ensuring the diversity and quality of the data.
During the pairing of data segments and annotation texts, the researchers used a series of sensor data as descriptive texts to precisely mark the start and end times of each action. This process employed multimodal fusion techniques, combining video, IMU data, and other sensor information to provide a comprehensive representation of actions. Although this method may introduce complex pairing and processing requirements, it accurately reflects the relationship between multimodal sensor data and actual actions.
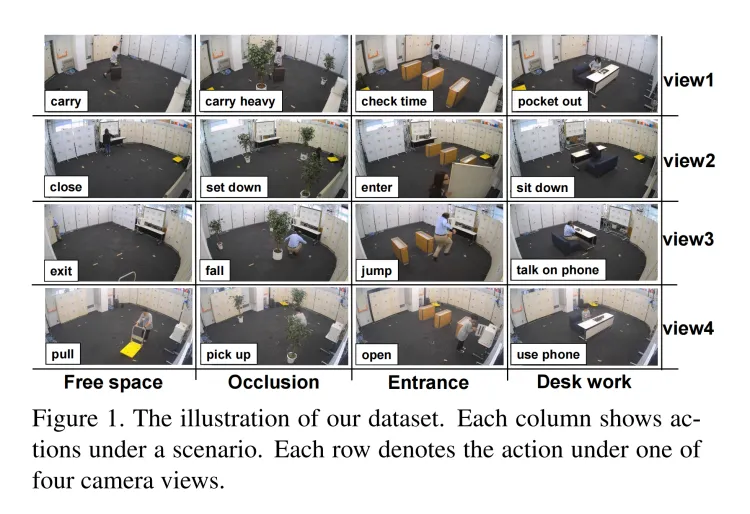
THUMOS
- Download Address: http://www.thumos.info/home.html
- Release Date: 2013-2015
- Description: The THUMOS Challenge is an annual competition aimed at academia and industry, designed to advance the field of video analysis, particularly in action recognition and action detection technologies. This challenge utilizes the THUMOS dataset for evaluation, which includes the following versions:
- THUMOS’13: This is the first version of the series, introduced as part of the challenge, primarily used for action classification tasks. The dataset contains a large number of sports action categories and is constructed using YouTube videos.
- THUMOS’14: In this version, in addition to action classification tasks, action detection tasks were introduced. The dataset includes validation and test sets, with annotations marking the time intervals during which actions occur in each video. THUMOS’14 covers 101 action categories and provides precise temporal boundaries for detection tasks.
- THUMOS’15: This version largely retains the data and task settings of THUMOS’14 but improves the quality of video annotations and the evaluation methods of the challenge to further advance research in action detection. THUMOS’15 contains over 430 hours of video data and 45 million frames, which are now publicly available. It includes the following components:
Training Set: Over 13,000 temporally trimmed video clips from 101 action categories.
Validation Set: Over 2,100 temporally untrimmed videos with temporal annotations for actions.
Background Set: Approximately 3,000 related videos guaranteed not to contain any instances of the 101 actions.
Test Set: Over 5,600 temporally untrimmed videos with hidden ground truth annotations.
For researchers working on video action recognition and detection, the THUMOS dataset provides a standardized testing platform, fostering algorithm innovation and improvement. Due to its precise annotations and diverse action categories, it is commonly used as a benchmark in academic research and algorithm development.
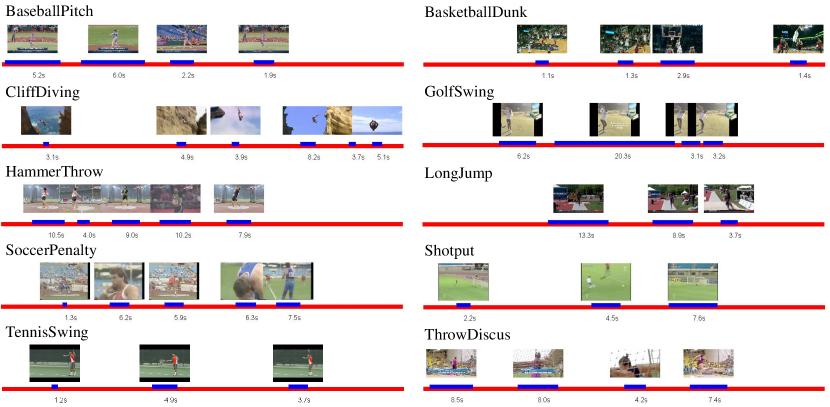
Multi-THUMOS
- Download Address: https://ai.stanford.edu/~syyeung/everymoment.html
- Paper Address: https://arxiv.org/abs/1507.05738
- Release Date: 2016
- Description: Multi-THUMOS is an extended and enhanced version of the original THUMOS dataset, specifically designed for multi-label action detection tasks. It builds upon traditional single-label action detection tasks by introducing multi-label annotations, allowing multiple action labels to be assigned to the same time segment. This enhancement presents a more challenging scenario for real-world video analysis, as multiple actions often occur simultaneously in practical settings. Compared to single-label datasets, Multi-THUMOS better reflects real-world complexities, improving the adaptability of models to intricate scenarios. Multi-THUMOS introduces new challenges to the field of video understanding, encouraging researchers to develop more sophisticated and robust multi-label detection models. This advancement not only drives technological progress but also provides a stronger foundation for various practical applications.
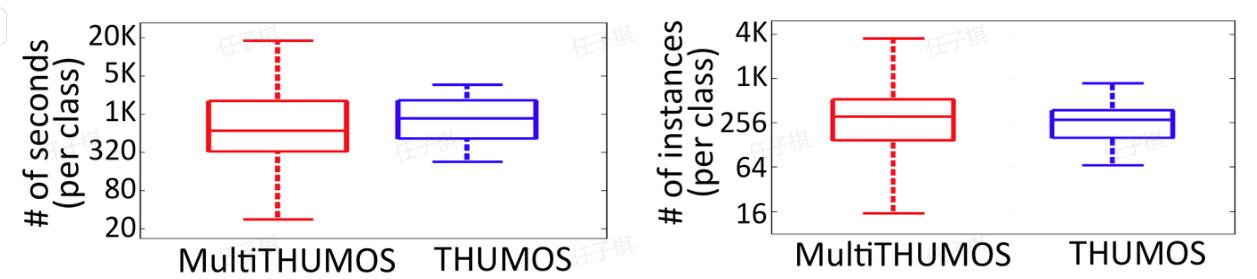
AVA Actions
- Publisher: Google, UC Berkeley
- Download Address: https://research.google.com/ava/
- Release Date: 2018
- Description: AVA Actions is a pioneering large-scale video action dataset designed to advance research in video understanding and temporal action recognition. This dataset contains annotations of actions performed by multiple individuals, covering a wide variety of daily life activities and interactive behaviors. The uniqueness of AVA Actions lies in its focus on high-quality temporal action annotations: each video segment is precisely annotated with actions performed by individuals at specific frames, ranging from simple actions like walking to complex multi-person interactions.
The research team collected numerous dynamic scenes from YouTube movies and refined specific action categories by filtering for "action tasks" in major categories, with a focus on activities involving human behavior and environmental interactions. Subsequently, they extracted relevant clips from publicly available movie videos and applied meticulous annotation standards to label each action frame by frame.
During the video segment-action label pairing phase, researchers precisely matched each action category with the corresponding video frames, ensuring high accuracy in temporal and action annotations. This process employed a comprehensive manual annotation approach, combining action classification and quantitative evaluation to provide a precise benchmark for action recognition. Although this method requires significant human effort and time, it accurately reflects the details of human actions in complex natural scenes.
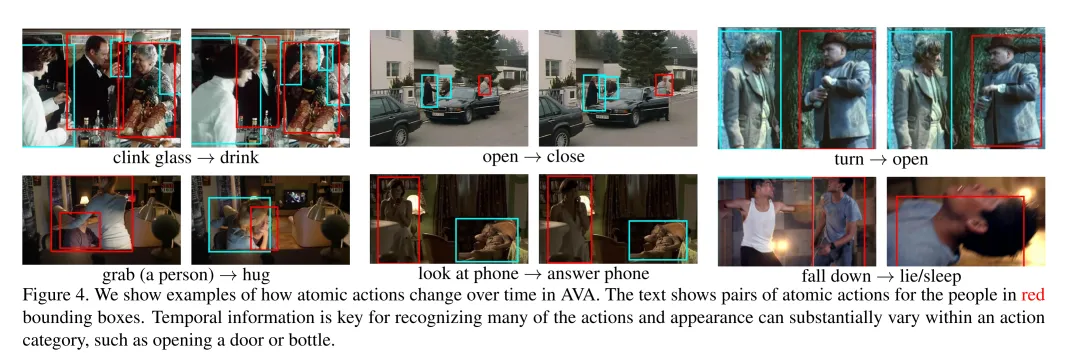
HMDB51
- Publisher: Brown University Computer Vision Team
- Download Address: https://serre-lab.clps.brown.edu/resource/hmdb-a-large-human-motion-database/
- Paper Address: https://ieeexplore.ieee.org/document/6126543
- Release Date: 2011
- Description: The HMDB51 dataset consists of 6,766 video clips from 51 action categories, with each category containing at least 101 clips. The initial evaluation scheme uses three different training/testing splits. In each split, there are 70 clips for training and 30 clips for testing per action category. The average accuracy across these three splits is used to measure the final performance. The dataset is small in size, making it convenient to download and use; the resolution is 320*240, and the complete dataset size is approximately 2GB. It comprises a large collection of real-world videos from various sources, including movies and web videos.The actions are primarily divided into five categories:
- General facial actions (smiling, laughing, etc.);
- Facial manipulations with object manipulations (smoking, eating, drinking, etc.);
- General body movements (cartwheels, clapping, climbing stairs, etc.);
- Interactions with objects (combing hair, golfing, horseback riding, etc.);
- Human-to-human actions (fencing, hugging, kissing, etc.).
Sports-1M
- Publisher: Google, Stanford University Department of Computer Science
- Download Address: https://github.com/gtoderici/sports-1m-dataset/
- Paper Address: https://ieeexplore.ieee.org/document/6909619
- Release Date: 2014
- Description: The Sports-1M dataset consists of over one million videos sourced from YouTube. It includes more than 1.1 million video clips, totaling over 5,000 hours of footage. Videos are automatically labeled using the YouTube Topics API by analyzing text metadata associated with the videos (e.g., tags, descriptions). The dataset covers 487 different types of sports activities, including football, basketball, swimming, gymnastics, and more. Each category contains between 1,000 and 3,000 videos. Each video is assigned one or more sports category labels, which are automatically generated based on the main content of the video, with approximately 5% of videos annotated with multiple class labels.
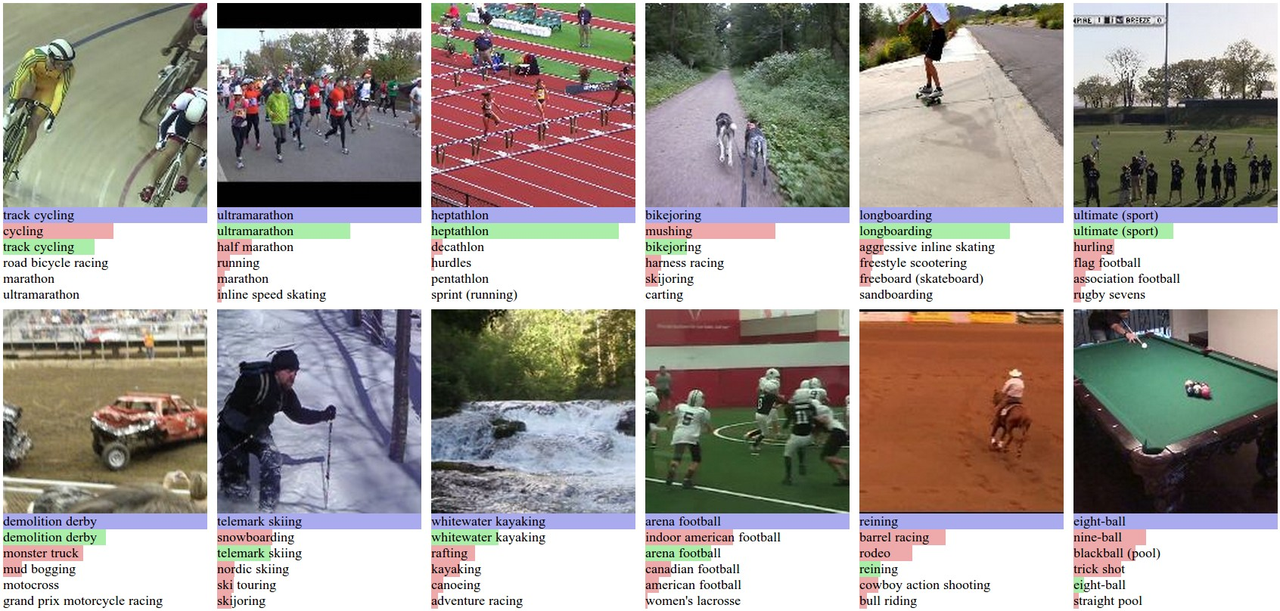
Conclusion
Action recognition video datasets, as critical resources in the field of AI vision, provide abundant material and precise guidance for model training. They drive continuous breakthroughs in action recognition technology, enabling widespread and in-depth applications across numerous domains. These datasets continually expand the boundaries of artificial intelligence, allowing machines to more intelligently understand and integrate into the world of human behavior. This lays a solid foundation for building a smarter, more convenient, and safer societal environment.