Nearly 300,000 downloads! PIN-14M: The New "Treasure House" of Multimodal Pre-training is Here!
Introduction to the PIN-14M Dataset
PIN-14M Project Link: https://huggingface.co/datasets/m-a-p/PIN-14M
The PIN (Paired and INterleaved) dataset is an innovative multimodal dataset format developed by the M-A-P team and open-source organizations like 2077AI. It aims to address the perceptual and reasoning errors present in existing multimodal datasets when training large multimodal models (LMMs). By integrating Markdown files and images, the PIN dataset is knowledge-intensive, scalable and supports diverse training strategies, significantly enhancing models' ability to learn complex tasks.
To tackle the challenges in training large multimodal models, particularly in interpreting complex visual data and inferring multimodal relationships, the M-A-P team has released the open-source dataset PIN-14M. PIN-14M contains 14 million samples, covering a wide range of scientific and web-based content, with a strong emphasis on data quality and ethical integrity. Preliminary results of the PIN-14M dataset validation demonstrate the immense potential of the PIN format in improving the performance of large multimodal models (LMMs).
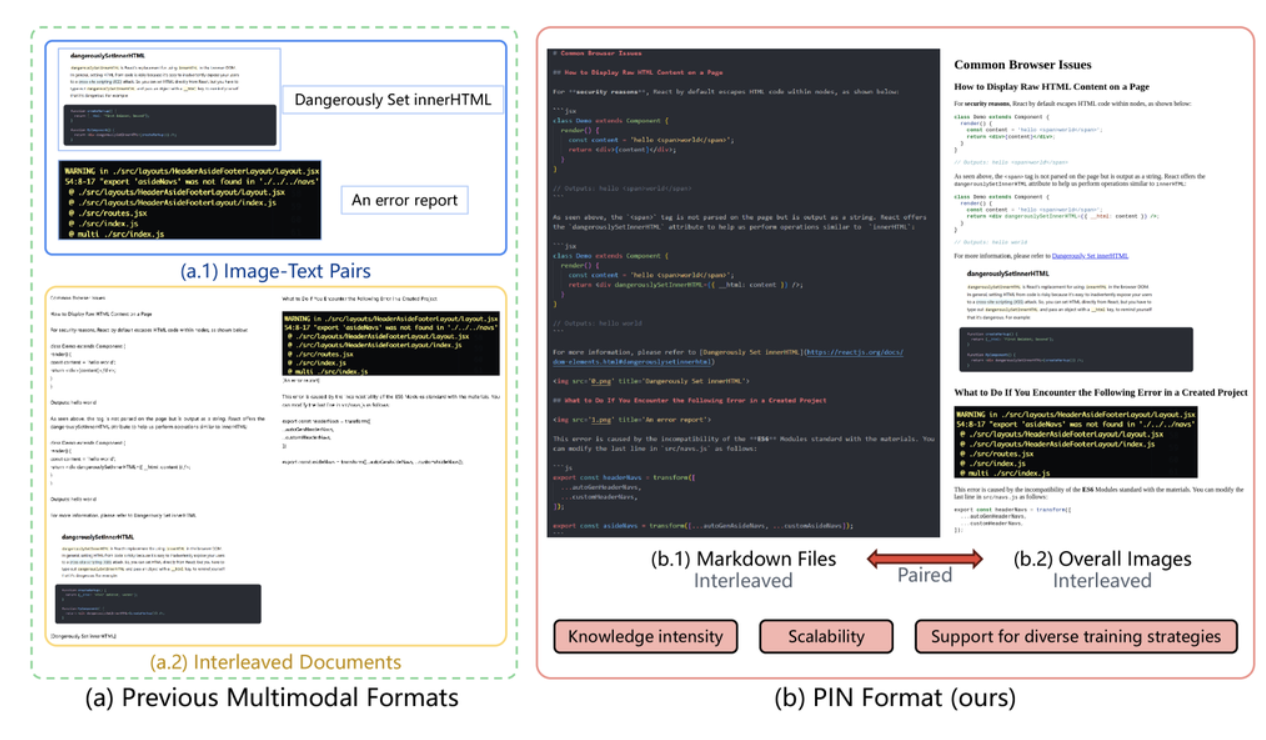
The PIN-14M development team is jointly comprised of the M-A-P team and the 2077AI open-source community. The M-A-P team is renowned for its cutting-edge contributions in the field of multimodal data research, focusing on advancing AI-driven solutions through creating powerful and diverse datasets. The 2077AI open-source community is dedicated to the standardization of AI data and the construction of its ecosystem. The collaboration between these two entities combines technological innovation with strategic vision. The release of PIN-14M as an open-source resource not only drives progress in the open-source domain but also reflects the team's commitment to fostering a more efficient and thriving AI data ecosystem. Together, they will continue to achieve groundbreaking advancements, enlarging the boundaries of what's possible in LMMs.
Construction of the PIN-14M Dataset
PIN-14M is built on three core principles: Knowledge-Intensive Design, Scalability, and Support for Multiple Training Strategies.
- Knowledge-Intensive Design: Each sample in PIN-14M features a tight integration of text and images, leveraging Markdown-formatted documents alongside overall images to fully express multimodal information. Additionally, the text portion utilizes markup elements such as bold, italics, and headings to structure knowledge hierarchically, helping models understand the structural relationship between different information.
- Scalability: The PIN dataset is designed with a unified format that can seamlessly integrate and convert existing multimodal datasets. Whether the original datasets are text-word pairs or interleaved documents, they can be easily transformed into the PIN format through a streamlined processing pipeline. This enables the construction of more comprehensive datasets.
- Support for Multiple Training Strategies: The PIN format is versatile, supporting a variety of training strategies such as image-text pairing, interleaved training, and other multimodal approaches. This flexibility allows models to learn from multiple perspectives, enhancing their reasoning capabilities and improving performance in complex scenarios.
To achieve these goals, the M-A-P team implemented a series of processing pipelines:
- Data Collection and Cleaning: The team gathered text and image data from diverse sources, including academic papers, web resources, and specialized platforms like arXiv and PMC.
- Data Formatting and Unification: The raw text and image data were converted and standardized into structured Markdown format, with overall images generated based on document content.
Additionally, to assure quality, each data entry was embedded with quality signals, allowing researchers to selectively filter data based on their needs. All data adheres to open-source licensing agreements, ensuring transparency and ethical compliance.
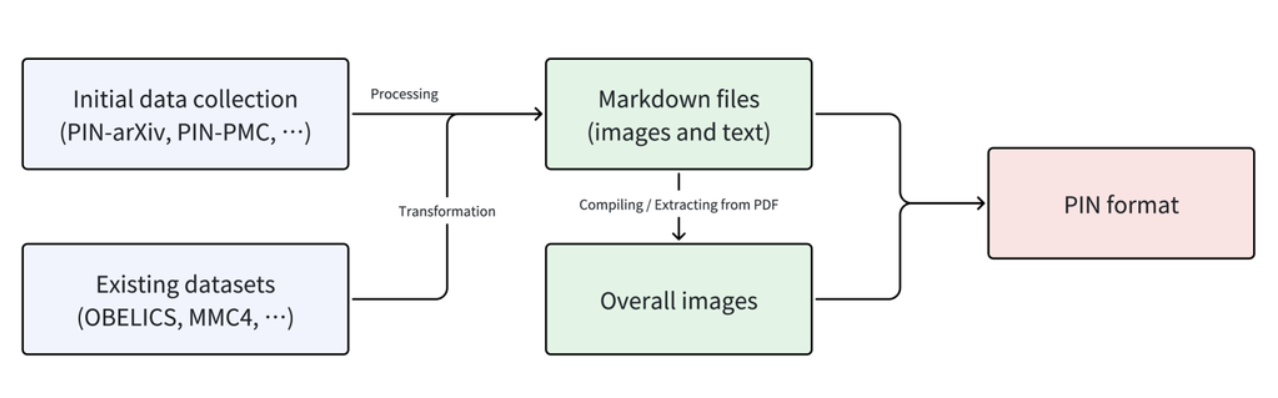
New Paradigm for Constructing Multimodal Training Datasets
The construction of the PIN data format and the open-sourcing of the PIN-14M dataset have provided a new paradigm for training multimodal large models. This is primarily reflected in the diversity, scalability, and compatibility brought by knowledge density and structured text, further enhancing the reasoning capabilities of trained models for complex tasks.
Traditional datasets typically focus on simple perceptual tasks (such as image classification, object detection, etc.), while the PIN dataset, through its complex knowledge structures and interleaved information layouts, helps models improve their abilities in reasoning, understanding, and interpreting complex multimodal relationships. Particularly in high-level tasks involving chart reasoning, scientific literature analysis, and similar challenges, the advantages of the PIN dataset are especially pronounced. This is attributed to the rich training data sources and the structured, high-density data format of PIN.
Unlike traditional image-text pair datasets, the PIN dataset not only includes simple pairings of images and text but also structures textual information through Markdown files. The Markdown format allows for semantic tagging of text, which is crucial for models to understand hierarchical relationships and key information within the text. The global images in the PIN format not only preserve the visual information of the images but also help models comprehend the deep connections between images and text. Compared to previous datasets, the PIN dataset integrates the layout information of entire pages into the images, enabling models to learn the overall structure and interrelationships within the images. In this way, the PIN dataset provides models with a more profound and systematic knowledge representation, enhancing their reasoning capabilities.
The PIN dataset covers not only traditional web data but also a wide range of academic literature, technical documents, and other professional domain content. The diversity of data sources makes the PIN dataset more broadly applicable in training large multimodal models, especially in tasks requiring reasoning and interpreting complex scientific and technical problems. The PIN dataset can provide more challenging samples in such scenarios.
Furthermore, the design of the PIN dataset takes into account the conversion and compatibility issues of existing multimodal datasets. By offering clear data format conversion processes, the PIN dataset can easily transform other datasets into the PIN format, thereby facilitating the expansion and integration of multimodal datasets. This feature is of significant importance for advancing the training of future multimodal models.
The introduction of the PIN dataset not only provides a new paradigm for constructing multimodal training datasets but also offers richer resources for training large AI models. By enhancing the knowledge density, scalability, and diversity of datasets, the PIN dataset holds immense potential for the future development of AI technologies. Due to HF's limitations, the project team will currently open-source the PIN-100M dataset, while the M-A-P team and the 2077AI team continue to produce more data, aiming to drive progress in the open-source ecosystem. In the future, with further expansion and optimization of the dataset, the PIN dataset is expected to become a core foundation for multimodal model training, advancing the application of AI in more complex tasks.