The Most Comprehensive Sharing for Embodied Intelligence Dataset: High-Quality Embodied Intelligence Datasets with Global Availability
As 2024 passes by in the blink of an eye, artificial intelligence has flourished at the levels of models, computing power, and data, gradually evolving from virtual interactions to physical implementations. With the advancement of large models and robotics technology, Embodied AI has endowed artificial intelligence systems with physical forms to enable interaction and learning with the environment. From motion programming to human teleoperation, from robotic arms to dexterous hands, and from Silicon Valley to China, Embodied AI has progressively established a developmental paradigm at both software and hardware levels.
Drawing lessons from the development path of autonomous vehicles, data is equally crucial for Embodied AI. Data not only serves as "fuel" to drive agents' perception and understanding of the environment but also helps construct environmental models and predict changes through multimodal sensors (such as vision, hearing, and touch). This enables agents to perform situational awareness and predictive maintenance based on historical data, thereby making better decisions. Building high-quality and diverse perception datasets is an indispensable foundational task. These datasets not only provide rich material for algorithm training but also serve as benchmark references for evaluating embodied performance.
Integer Smart has always been committed to becoming the "data partner of the artificial intelligence industry." As we move forward, let us take a look at the high-quality Embodied AI datasets available globally.
1. AgiBot World
- Publisher: Zhiyuan Robotics, Shanghai AI Laboratory, etc.
- Release Date: December 2024
- Project Links:
- HuggingFace: https://huggingface.co/agibot-world
- Github: https://github.com/OpenDriveLab/agibot-world
- Project Homepage: https://agibot-world.com/
- Dataset Link: https://huggingface.co/datasets/agibot-world/AgiBotWorld-Alpha
- Description: Zhiyuan Robotics, in collaboration with the Shanghai AI Laboratory, the National-Local Joint Humanoid Robot Innovation Center, and Shanghai Cooperas, has officially open-sourced the AgiBot World project. AgiBot World is the world's first million-scale real-world dataset based on comprehensive real-world scenarios, versatile hardware platforms, and full-process quality control. The scenarios covered in the AgiBot World dataset are diverse and multifaceted, ranging from basic operations such as grasping, placing, pushing, and pulling, to complex actions like stirring, folding, and ironing, encompassing almost all scenarios required for daily human life.
- Scale: Contains over 1 million demonstration trajectories from 100 robots. In terms of long-term data scale, it exceeds Google's OpenX-Embodiment dataset by ten times. Compared to Google's open-sourced Open X-Embodiment dataset, AgiBot World's long-term data scale is 10 times larger, the scope of scenarios is 100 times broader, and the data quality has been elevated from laboratory-grade to industrial-grade standards.
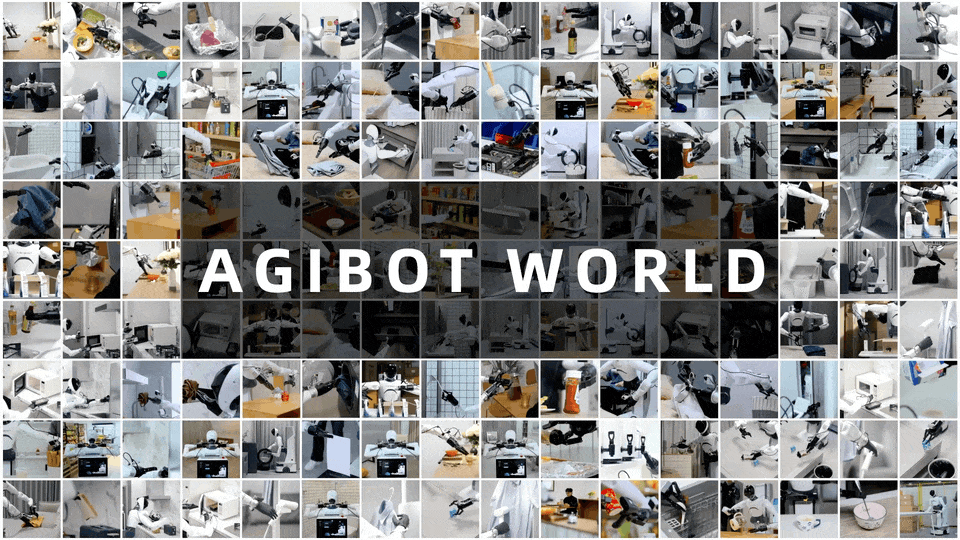
AgiBot World
- Scenarios: The AgiBot World dataset was created in Zhiyuan's self-built large-scale data collection factory and application experimental base, with a total area of over 4,000 square meters. It includes more than 3,000 real-world items. On one hand, it provides a venue for large-scale robot data training, and on the other hand, it accurately replicates five core scenarios: home, dining, industrial, commercial, and office environments. This comprehensively covers the typical application needs of robots in both production and daily life.
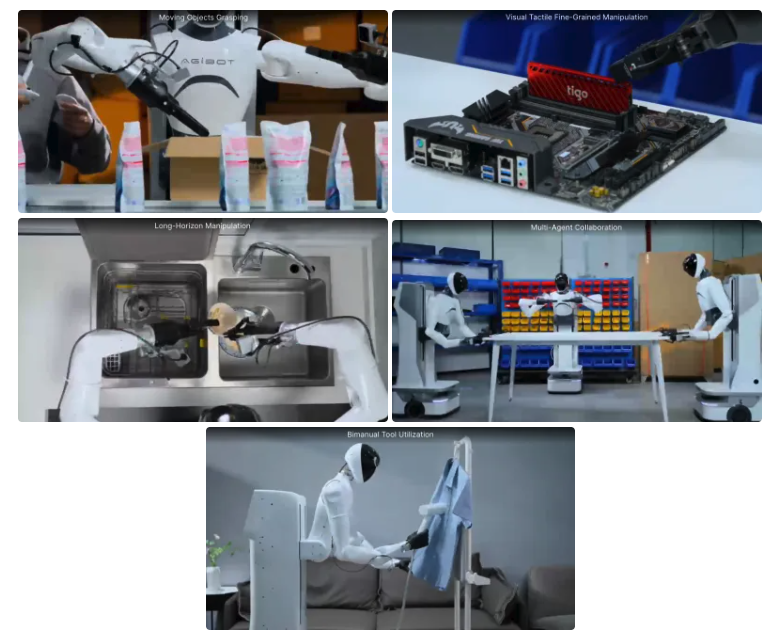
Diversified Task Demonstration
- Skills: The AgiBot World dataset covers hundreds of general scenarios, including home (40%), dining (20%), industrial (20%), office (10%), and supermarket (10%), as well as over 3,000 operational objects. Compared to the widely used Open X-Embodiment and DROID datasets abroad, the AgiBot World dataset significantly improves in terms of data duration distribution. Among its tasks, 80% are long-duration tasks, with task durations concentrated between 60s and 150s. It also includes multiple atomic skills, with long-duration data being more than 10 times that of DROID and OpenX-Embodiment. The over 3,000 items essentially cover these five major scenarios.
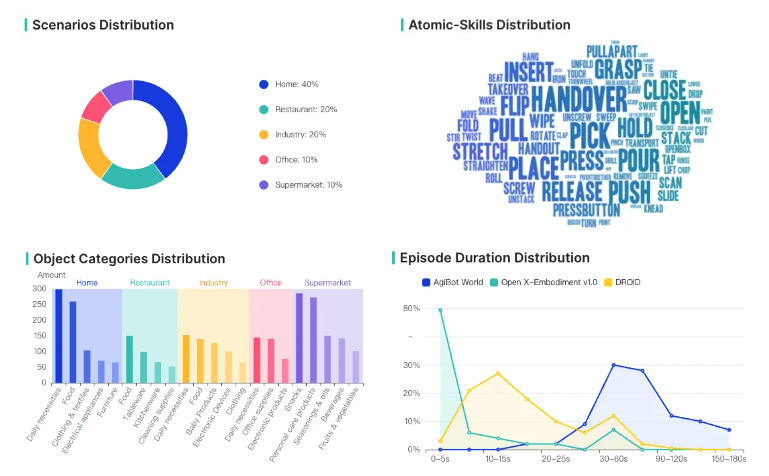
Distribution of Agibot World Dataset
- Data Collection: AgiBot World is based on data collected using a fully controllable mobile dual-arm robot, equipped with advanced devices such as visual-tactile sensors, six-dimensional force sensors, and six-degree-of-freedom dexterous hands. This setup is suitable for cutting-edge research in imitation learning and multi-agent collaboration. The Zhiyuan Genie-1 robot includes 8 surround-layout cameras for real-time 360-degree omnidirectional perception, a six-degree-of-freedom dexterous hand with a six-dimensional force sensor and high-precision tactile sensor at the end, and a total of 32 active degrees of freedom across the entire body.
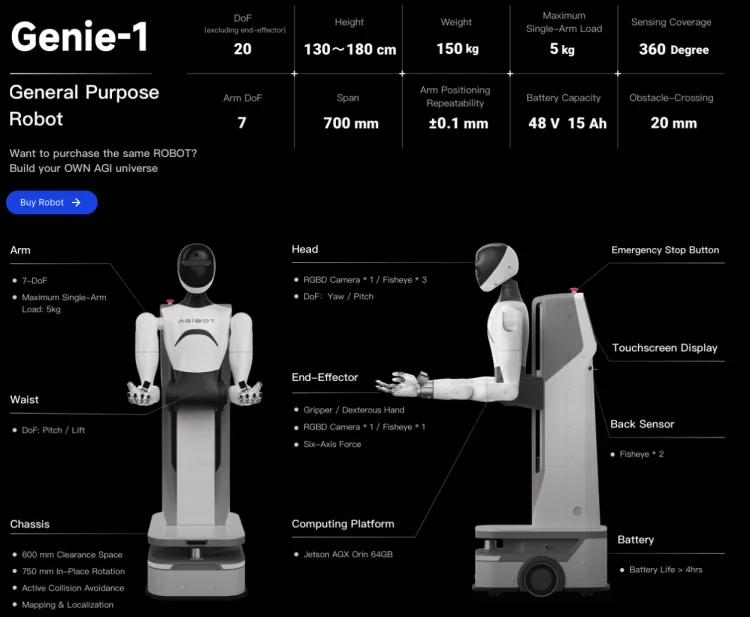
Zhiyuan Robot
2. Open X-Embodiment
- Publisher: Google DeepMind and 21 other global institutions
- Release Date: October 2023
- Project Link: https://robotics-transformer-x.github.io/
- Paper Link: https://arxiv.org/abs/2310.08864
- Dataset Link: https://github.com/google-deepmind/open_x_embodiment
- Description: Open X-Embodiment is an open, large-scale, standardized robotics learning dataset created by Google DeepMind in collaboration with 34 research labs from 21 internationally renowned institutions. It integrates 60 existing robotics datasets. The Open X-Embodiment Dataset researchers have converted datasets from different sources into a unified data format, making it easy for users to download and use. Each set of data is presented as a series of "episodes" and described using the RLDS format established by Google, ensuring high compatibility and ease of understanding.
- Scale: It covers 22 different types of robots, ranging from single-arm robots to dual-arm robots and quadrupedal robots, and includes over 1 million robot demonstration trajectories, 311 scenarios, 527 skills, and 160,266 tasks.
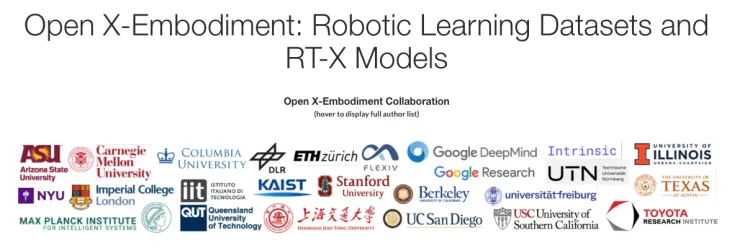
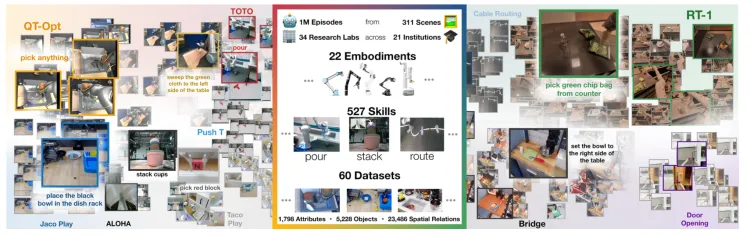
Open X-Embodiment: Robotic Learning Datasets and RT-X Models
- Scenarios: Researchers trained two models on the mixed robot data:
- RT-1, an efficient Transformer-based architecture specifically designed for robot control;
- RT-2, a large vision-language model jointly fine-tuned to output robot actions as natural language tokens.
Both models output robot actions represented relative to the robot gripper frame. The robot action is a 7-dimensional vector consisting of x, y, z, roll, pitch, yaw, and gripper opening, or the rates of these quantities. For datasets where the robot does not use certain dimensions, the corresponding dimension values are set to zero during training. The RT-1 model trained on the mixed robot data is referred to as RT-1-X, and the RT-2 model trained on the mixed robot data is referred to as RT-2-X.
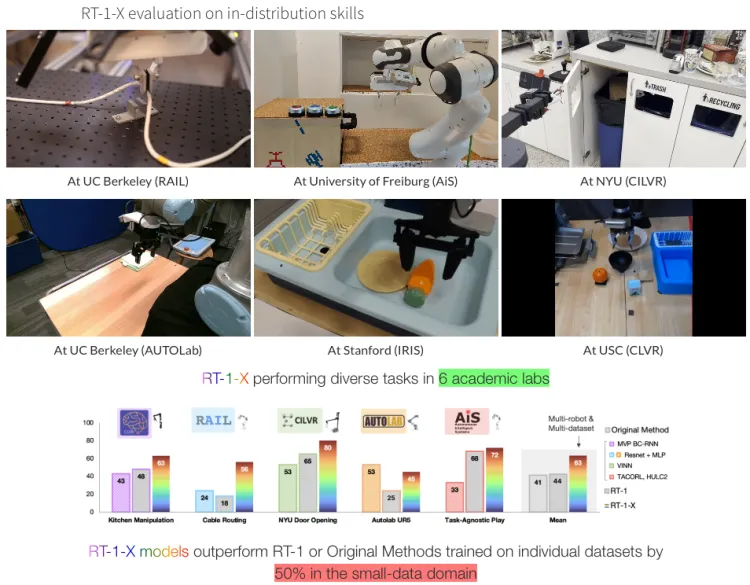
RT-1-X outperforms RT-1 trained on a single dataset or the original methods by 50% in the field of small data.
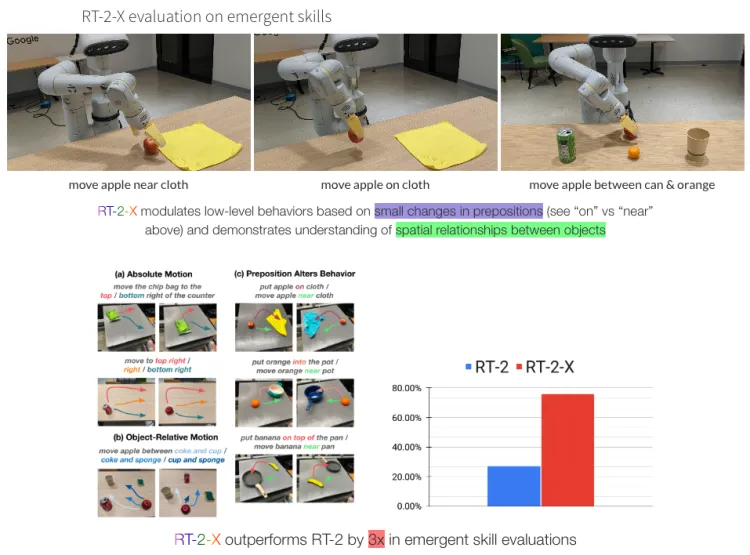
RT-2-X outperforms RT-2 by three times in emerging skill evaluation.
- Skills: Common task skills in the dataset include picking, moving, pushing, placing, etc. Task objectives include Shapes, Containers, Furniture, Food, Appliances, Utensils, etc.
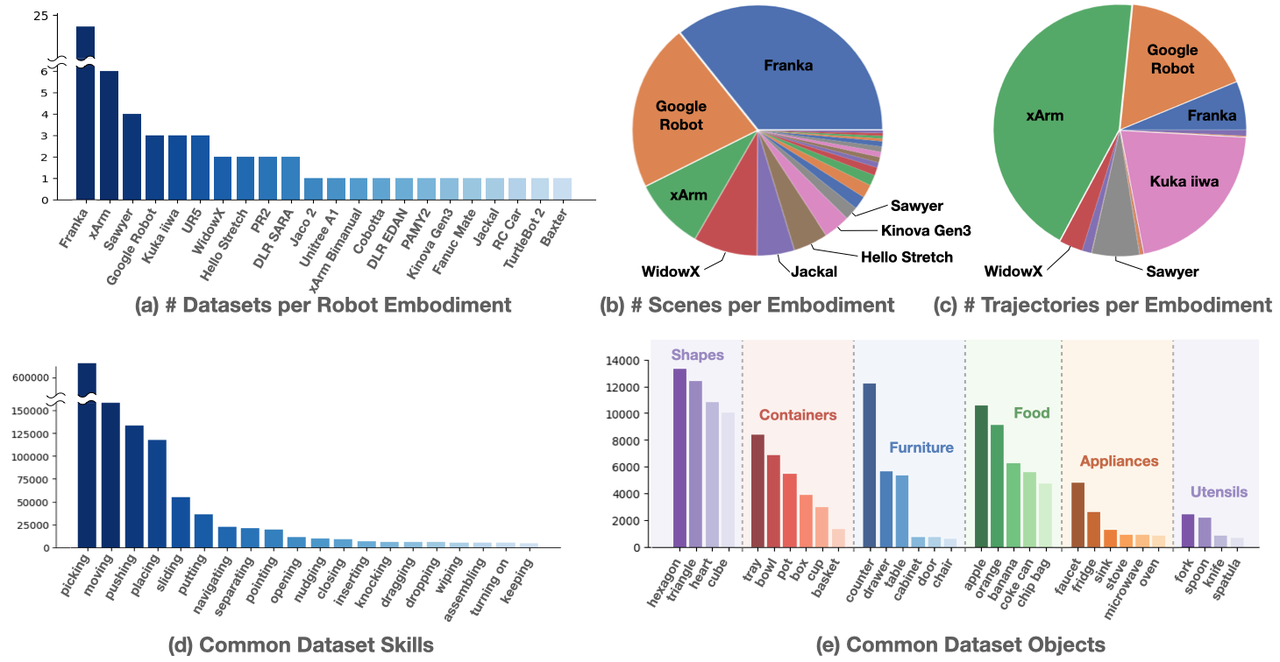
Open X-Embodiment Skill Distribution
- Data Collection: In terms of scene distribution, Franka robots dominate, followed by Google Robot and xArm. In terms of trajectory distribution, xArm contributes the most trajectories, followed by Google Robot, Franka, Kuka, iiwa, Sawyer, and WidowX.
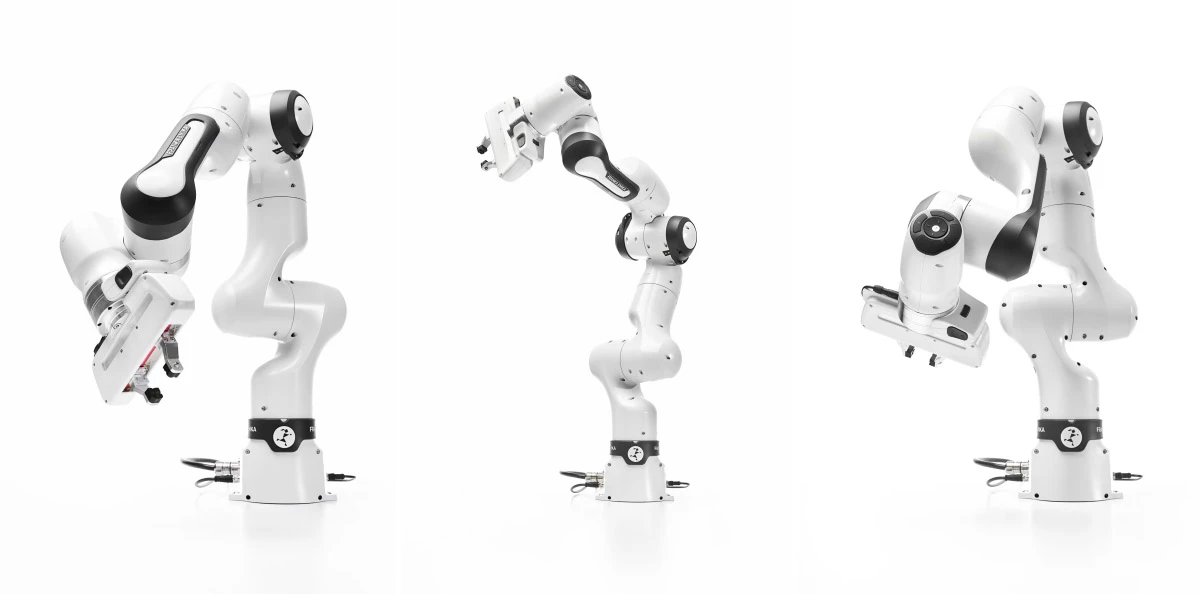
Franka Robotics
3. DROID
- Publisher: Stanford University, UC Berkeley, Toyota Research Institute, etc.
- Release Date: March 2024
- Project Link: https://droid-dataset.github.io/
- Paper Link: https://arxiv.org/abs/2403.12945
- Dataset Link: https://droid-dataset.github.io/
- Description: Creating large, diverse, and high-quality robot manipulation datasets is a crucial cornerstone for developing more powerful and robust robot manipulation strategies. However, creating such datasets is challenging: collecting robot manipulation data in diverse environments presents logistical and safety challenges and requires significant hardware and human resources. Researchers introduced DROID (Distributed Robot Interaction Dataset), a diverse robot manipulation dataset. Studies have shown that, compared to state-of-the-art methods utilizing existing large-scale robot manipulation datasets, DROID improves policy performance, robustness, and generalization by an average of 20%.
- Scale: The DROID dataset comprises 1.7TB of data, including 76,000 robot demonstration trajectories, covering 86 tasks and 564 scenarios.
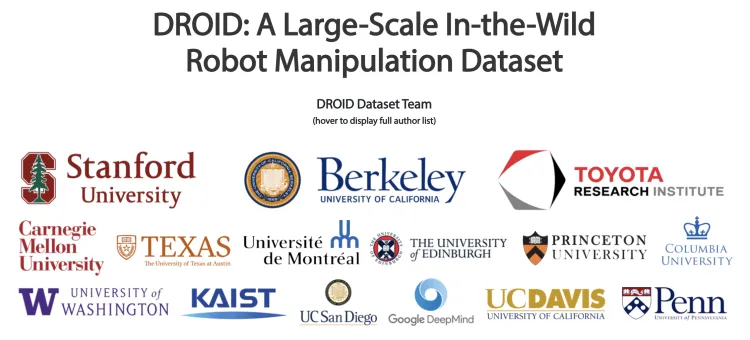
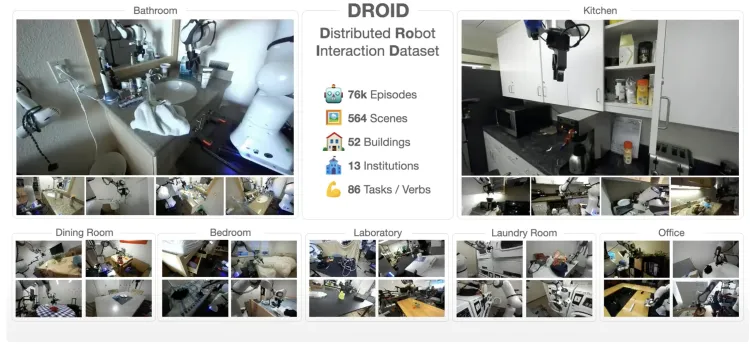
DROID: A Large-Scale In-the-Wild Robot Manipulation Dataset
- Scenarios: The dataset covers 564 demonstration scenarios, including industrial offices, home kitchens, industrial kitchens, offices, living rooms, corridors/closets, bedrooms, dining rooms, bathrooms, laundry rooms, and more.

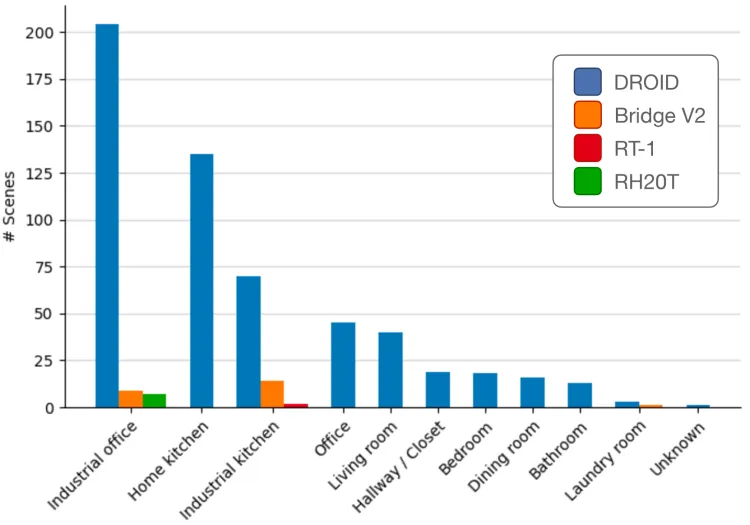
Oroid Dataset Scene Distribution
- Skills: The DROID dataset includes 86 action tasks, covering a broader range of scenario types and significantly more long-tail task distributions compared to previous datasets.
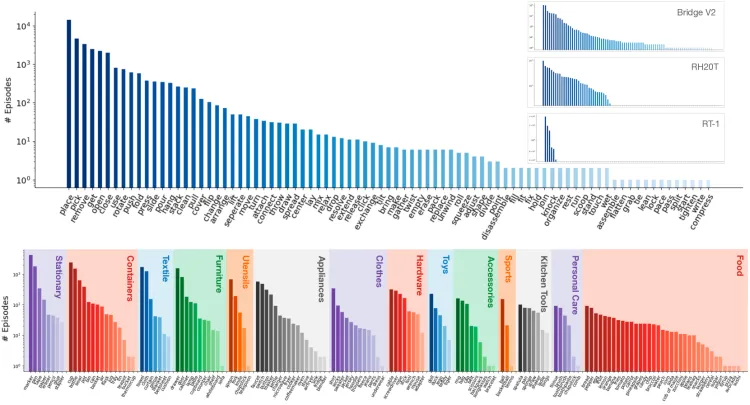
Oroid Dataset Skill Distribution
- Data Collection: DROID uses the same hardware setup across all 13 institutions to simplify data collection while maximizing portability and flexibility. The setup includes a Franka Panda 7DoF robot arm, two adjustable Zed 2 stereo cameras, a wrist-mounted Zed Mini stereo camera, and an Oculus Quest 2 headset with controllers for teleoperation. All equipment is mounted on a portable, height-adjustable table for quick scene switching.
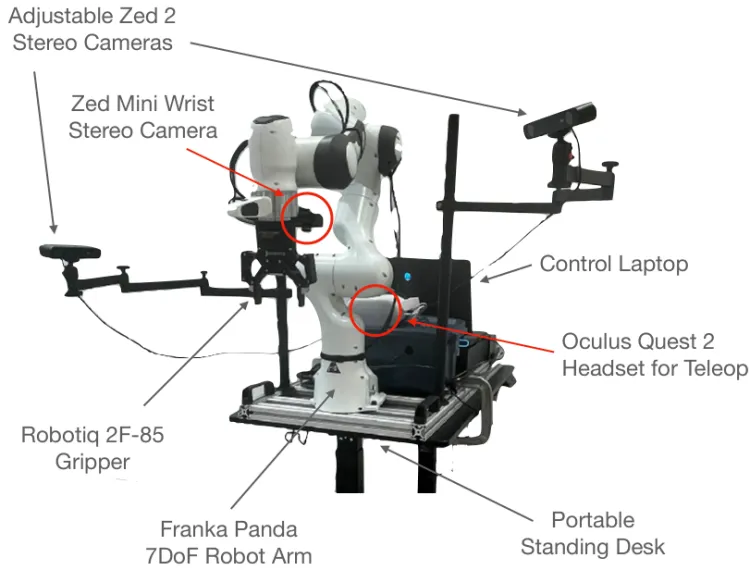
Droid Data Collection Device
4. RT-2/RT-1
- Publisher: Google DeepMind
- Release Date: December 2022
- Project Link: https://robotics-transformer2.github.io/
- Paper Link: https://robotics-transformer2.github.io/assets/rt2.pdf
- Dataset Link: https://github.com/google-research/robotics_transformer
- Description: In 2022, Google DeepMind's research team introduced a large-scale real-world robotics dataset, along with the multi-task model RT-1: Robotics Transformer. In July 2023, researchers proposed RT-2: Vision-Language-Action Models (VLA).
- Scale: The RT-2 dataset primarily consists of two major components. The first is the WebLI vision-language dataset, which contains approximately 10 billion image-text pairs covering 109 languages, filtered down to 1 billion training samples. The second component is the robotics dataset from RT-1 and others. RT-1 collected 130,000 episodes over a span of 17 months using 13 EDR robotic arms equipped with 7-degree-of-freedom arms, two-finger grippers, and mobile bases. The total data volume reached 111.06GB. Each episode not only includes the actual records of robot actions but also comes with corresponding human instruction text annotations.
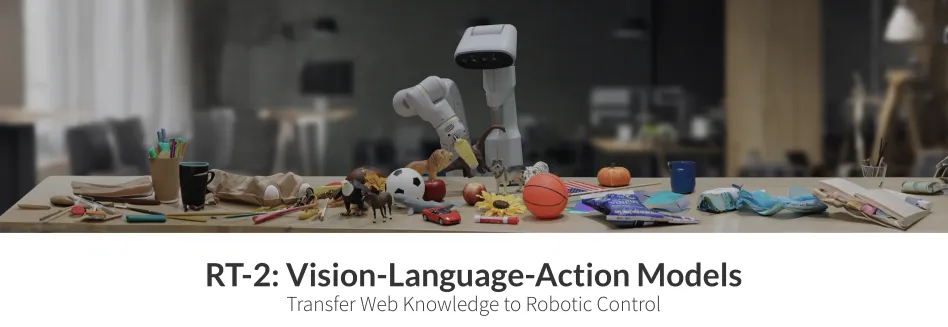
RT-2: Vision-Language-Action Models Transfer Web Knowledge to Robotic Control
- Scenarios and Skills: The RT-2 scenarios primarily focus on environments such as homes and kitchens, involving items like furniture, food, and utensils. The skills mainly include common operations such as pick-and-place, as well as more challenging skills like wiping and assembling. Tasks range from picking up and placing objects to more complex tasks such as opening and closing drawers, handling slender objects, pushing over objects, pulling napkins, and opening cans, covering tasks involving over 700 different objects.
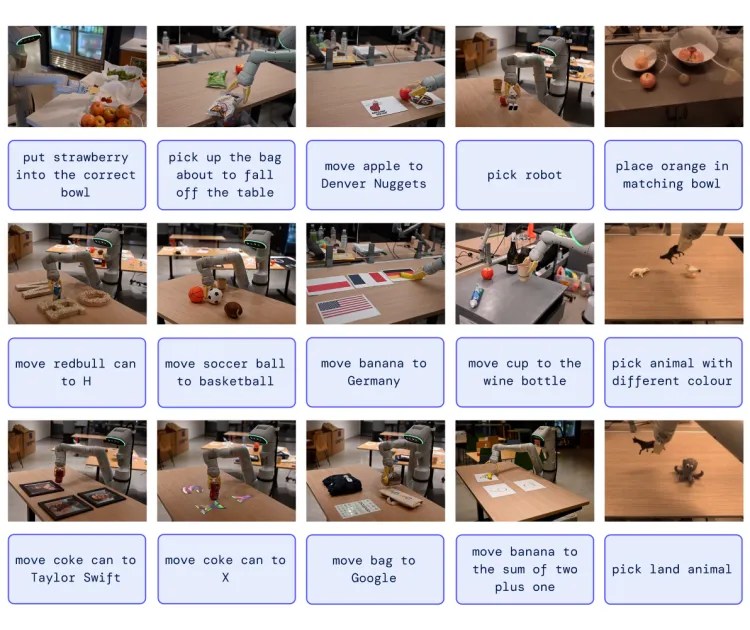
RT-2 Scene Task
- Research Significance: RT-2 improves performance on unseen scenarios from 32% in RT-1 to 62%, demonstrating the significant benefits of large-scale pre-training. It shows substantial improvements compared to baselines pre-trained on purely visual tasks, such as VC-1 and reusable representations for robotic manipulation (R3M), as well as algorithms using VLM for object recognition, such as Open-World Object Manipulation (MOO). RT-2 demonstrates that vision-language models (VLMs) can be transformed into powerful vision-language-action (VLA) models, which can directly control robots by combining VLM pre-training with robotic data.
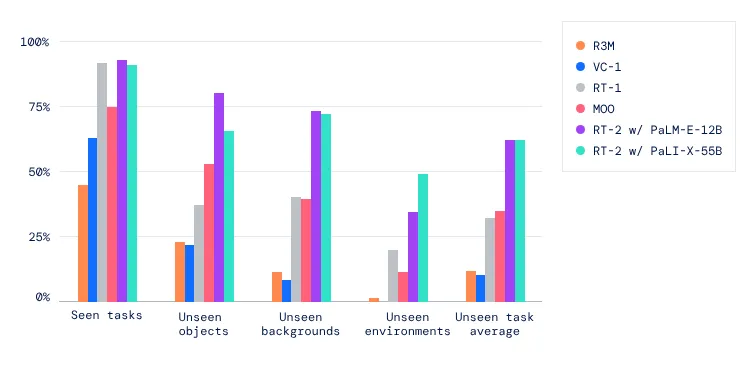
Performance Comparison of RT-2
5. BridgeData V2
- Publisher: UC Berkeley, Stanford, Google DeepMind, CMU
- Release Date: September 2023
- Project Link: https://rail-berkeley.github.io/bridgedata/
- Paper Link: https://arxiv.org/abs/2308.12952
- Dataset Link: https://rail.eecs.berkeley.edu/datasets/bridge_release/data/
- Description: BridgeData V2 is a large and diverse dataset of robotic manipulation behaviors, designed to promote research in scalable robot learning. The dataset is compatible with open-vocabulary, multi-task learning methods conditioned on goal images or natural language instructions. Skills learned from the data can generalize to new objects, environments, and institutions.
- Scale: The BridgeData V2 dataset contains 60,096 trajectories, of which 50,365 are teleoperated demonstrations and 9,731 are deployments via scripted pick-and-place policies.
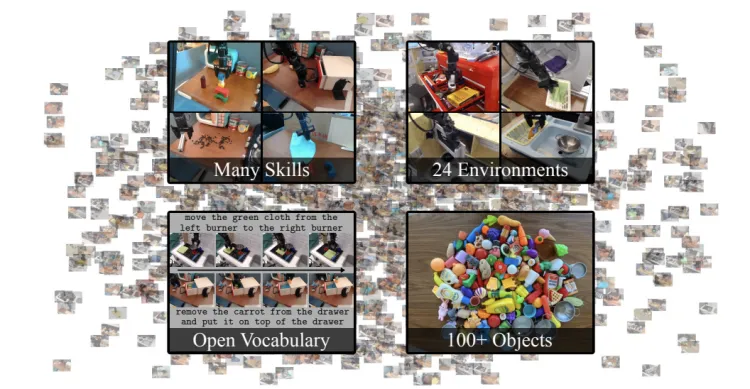
BridgeData V2: A Dataset for Robot Learning at Scale
- Scenarios: The 24 environments in BridgeData V2 are divided into 4 categories. Most of the data comes from 7 different toy kitchen setups, which include combinations of sinks, stoves, and microwaves. The remaining environments come from various sources, including different tabletops, standalone toy sinks, toy washing machines, and more.
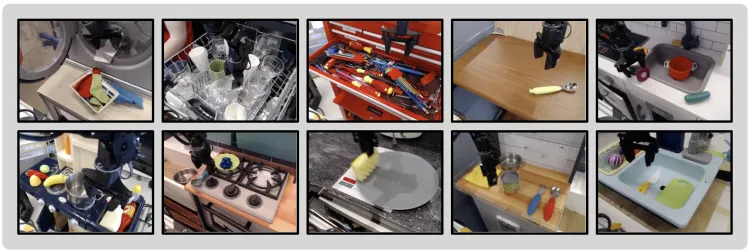
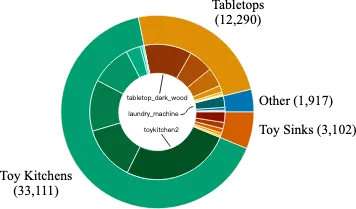
BridgeData V2 Setting
- Skills: The majority of the data comes from basic object manipulation tasks, such as picking and placing, pushing, and sweeping; some data comes from environment manipulation, such as opening and closing doors and drawers; and some comes from more complex tasks, such as stacking blocks, folding cloth, and sweeping granular media.
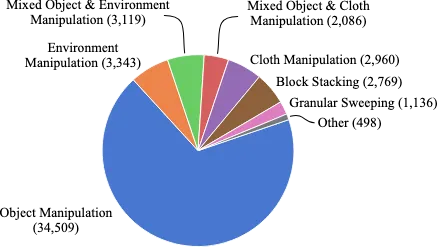
BridgeData V2 Skill
- Data Collection: Data was collected using a WidowX250 6-DOF robotic arm, with the robot being teleoperated using VR controllers at a control frequency of 5Hz. The average trajectory length is 38 time steps. Sensing was performed using an RGB-D camera fixed in an over-the-shoulder view, two RGB cameras with random poses during data collection, and an RGB camera attached to the robot wrist. Images were saved at a resolution of 640x480.
6. RoboSet
- Publisher: Carnegie Mellon University, FAIR-MetaAI
- Release Date: September 2023
- Project Link: https://robopen.github.io/
- Paper Link: https://arxiv.org/pdf/2309.01918.pdf
- Dataset Link: https://robopen.github.io/roboset/
- Description: The RoboSet dataset from the RoboAgent project is a large-scale, real-world, multi-task dataset collected from a series of daily household activities in kitchen environments. RoboSet consists of kinesthetic demonstrations and teleoperated demonstrations. The dataset includes multi-task activities, with four different camera views per frame, as well as scene variations for each demonstration.
- Scale: By training on just 7,500 trajectories, it demonstrates a general-purpose RoboAgent capable of exhibiting 12 manipulation skills across 38 tasks and generalizing them to hundreds of different unseen scenarios.
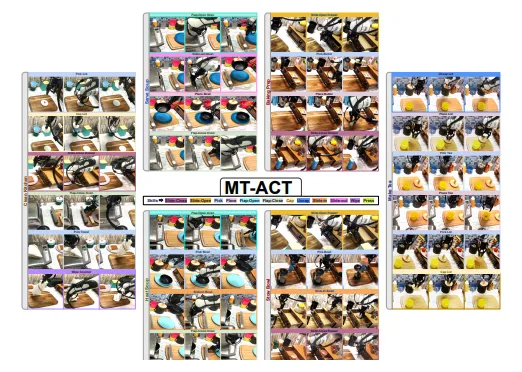
RoboAgent: Generalization and Efficiency in Robot Manipulation via Semantic Augmentations and Action Chunking
- Scenarios: The dataset primarily focuses on daily kitchen activity scenarios, such as making tea, baking, and similar tasks.
- Skills: RoboSet covers 12 manipulation skills across 38 tasks.
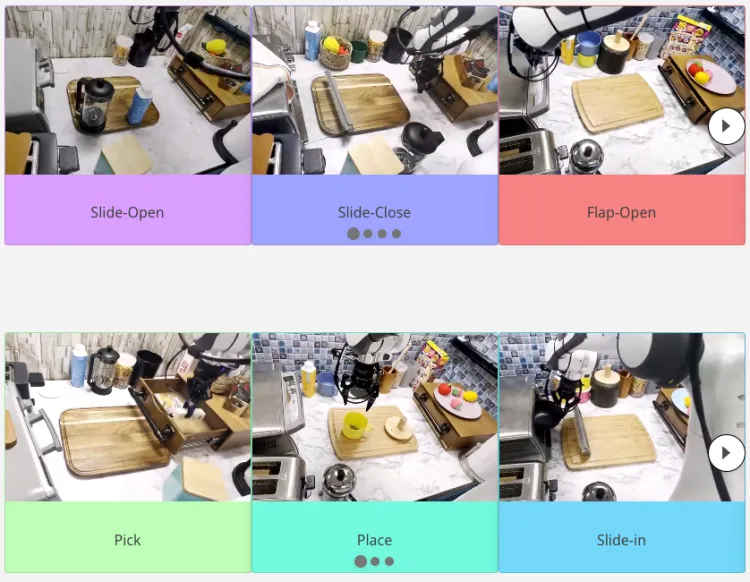
- Data Collection: Data was collected using a Franka-Emika robot equipped with a Robotiq gripper through human teleoperation. Daily kitchen activities were broken down into different subtasks, and corresponding robot data was recorded during the execution of these subtasks.
7. BC-Z
- Publisher: Robotics at Google, X (The Moonshot Factory), UC Berkeley, Stanford University
- Release Date: February 2022
- Project Link: https://sites.google.com/view/bc-z/
- Paper Link: https://arxiv.org/abs/2202.02005
- Dataset Link: https://www.kaggle.com/datasets/google/bc-z-robot
- Description: Researchers investigated how to enable vision-based robotic operating systems to generalize to new tasks, addressing this challenge from the perspective of imitation learning. To this end, they developed an interactive and flexible imitation learning system that can learn from demonstrations and interventions and can be conditioned on different forms of information conveying tasks, including pre-trained natural language embeddings or videos of humans performing tasks. When scaling data collection on real robots to over 100 different tasks, researchers found that the system could perform 24 unseen manipulation tasks with an average success rate of 44%, without requiring any robot demonstrations for these tasks.
- Scale: Contains 25,877 different manipulation tasks, covering 100 diverse tasks.
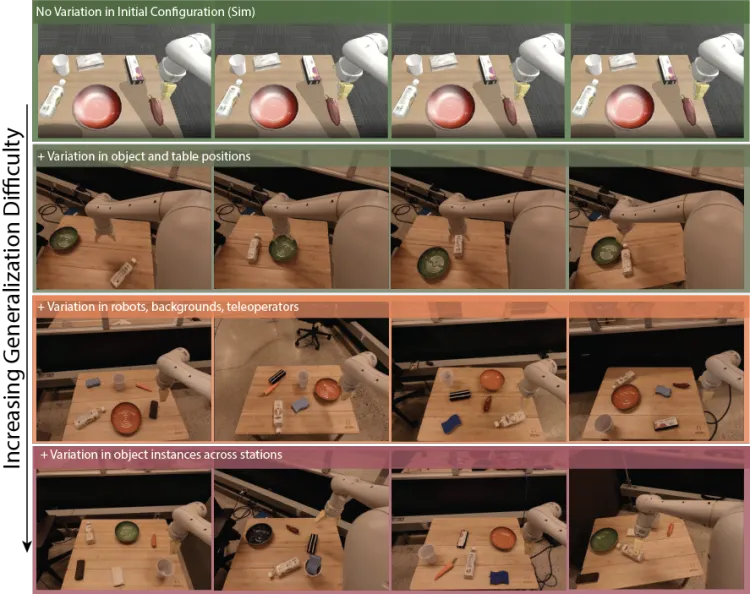
BC-Z: Zero-Shot Task Generalization with Robotic Imitation Learning
- Scenarios:
- Variations in object positions;
- Changes in scene backgrounds due to data collection across multiple locations;
- Subtle hardware differences between robots;
- Variations in object instances;
- Multiple distracting objects;
- Closed-loop, RGB-only visual motor control at 10Hz asynchronous inference, resulting in over 100 decisions per episode (i.e., long-term tasks that are challenging for sparse RL objectives).
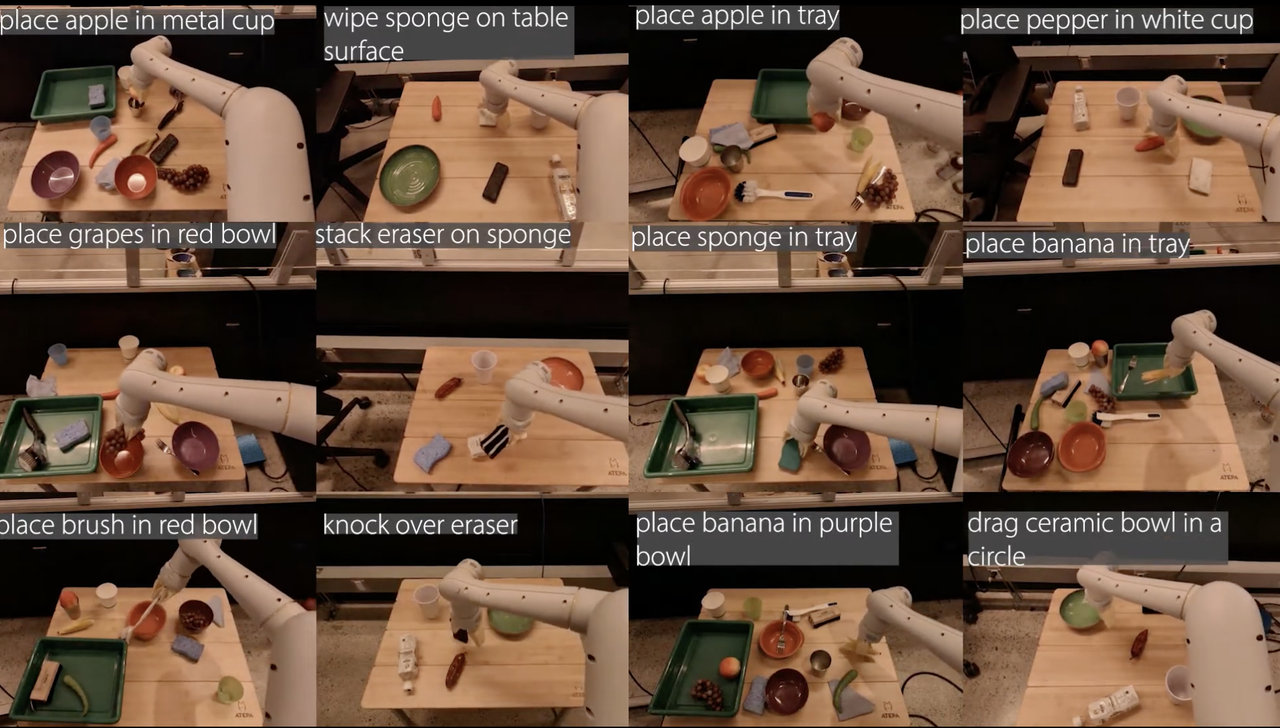
BC-Z Scene Task
8. MIME
- Publisher: The Robotics Institute, Carnegie Mellon University
- Release Date: October 2018
- Paper Link: https://arxiv.org/abs/1810.07121
- Description: In the fields of robotics learning and artificial intelligence, enabling robots to learn complex tasks by imitating human behavior is an important research direction. Traditional learning methods face limitations when dealing with complex tasks that require multiple interactive actions. The Multiple Interactions Made Easy (MIME) project aims to provide large-scale demonstration data to facilitate imitation learning for robots. By collecting rich demonstrations of human behavior, robots can learn various interactive actions, thereby better accomplishing complex tasks. The MIME project fills the gap in the field of robot imitation learning by providing large-scale demonstration data that includes multiple interactive actions.
- Scale: The MIME dataset contains 8,260 human-robot demonstrations across more than 20 different robot tasks. These tasks range from simple tasks like pushing objects to more challenging tasks like stacking household items, consisting of videos of human demonstrations and kinesthetic trajectories of robot demonstrations.
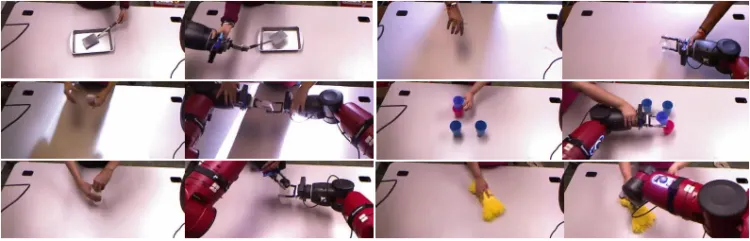
Multiple Interactions Made Easy (MIME): Large Scale Demonstrations Data for Imitation
- Skills: MIME pioneered methods for collecting demonstration datasets. The MIME project includes a large amount of demonstration data, which provides ample samples for robot learning. The rich sample size helps robots learn various situations and action patterns, enhancing their generalization capabilities and enabling them to better handle complex and variable tasks in real-world scenarios. The dataset is not limited to simple actions but covers a variety of interactive actions. For example, in a household scenario, a robot can learn a series of coherent actions such as picking up objects, placing objects, opening drawers, closing drawers, and placing items into drawers. This diverse interactive action data enables robots to understand the sequence and logical relationships of tasks.
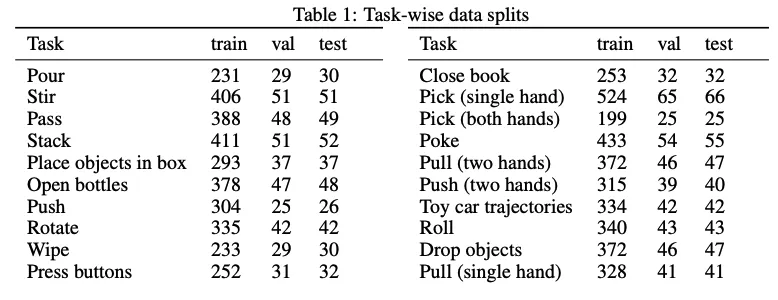
Mime Action Skills
- Data Collection: A Baxter robot in gravity-compensated kinesthetic mode was used. Baxter is a dual-arm manipulator with 7-DOF arms equipped with two-finger parallel grippers. Additionally, the robot is equipped with a Kinect mounted on its head and two SoftKinetic DS325 cameras, each mounted on the robot's wrists. The head camera acts as an external camera observing tasks on the table, while the wrist cameras serve as the robot's eyes, moving with the arms.

Multiple Views in Robot Demonstration
9. ARIO
- Publisher: Pengcheng Laboratory, Southern University of Science and Technology, Sun Yat-sen University, etc.
- Release Date: August 2024
- Project Link: https://imaei.github.io/project_pages/ario/
- Paper Link: https://arxiv.org/abs/2408.10899
- Dataset Link: https://openi.pcl.ac.cn/ARIO/ARIO_Dataset
- Description: The ARIO (All Robots In One) Embodied Intelligence Data Open-Source Alliance was jointly initiated by leading institutions such as Pengcheng Laboratory, AgileX Robotics, Sun Yat-sen University, Southern University of Science and Technology, and the University of Hong Kong. Pengcheng Laboratory's Embodied Intelligence Institute first designed a set of format standards for embodied big data. These standards can record control parameters for various forms of robots, feature a clear data organization structure, and are compatible with sensors of different frame rates while recording corresponding timestamps to meet the precise requirements of embodied intelligence models for perception and control timing.
- Scale: The ARIO dataset includes 258 scenarios, 321,064 tasks, and 3,033,188 demonstration trajectories. The data modalities cover 2D images, 3D point clouds, sound, text, and tactile data. The data comes from three main sources:
(1) real-world environment setups and tasks collected by humans;
(2) virtual scenes and object models designed using simulation engines like MuJoCo and Habitat, with robot models driven by simulation engines;
(3) analysis and processing of existing open-source embodied datasets, converting them into data that conforms to the ARIO format standards.
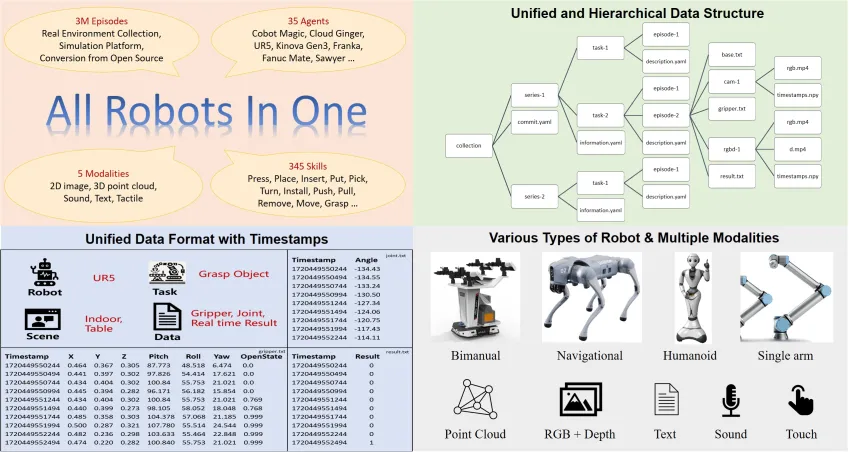
All Robots in One: A New Standard and Unified Dataset for Versatile, General-Purpose Embodied Agents
- Scenarios and Skills: ARIO's scenarios cover desktops, open environments, multi-room setups, kitchens, household chores, corridors, and more. Skills include pick, place, put, move, open, grasp, etc.
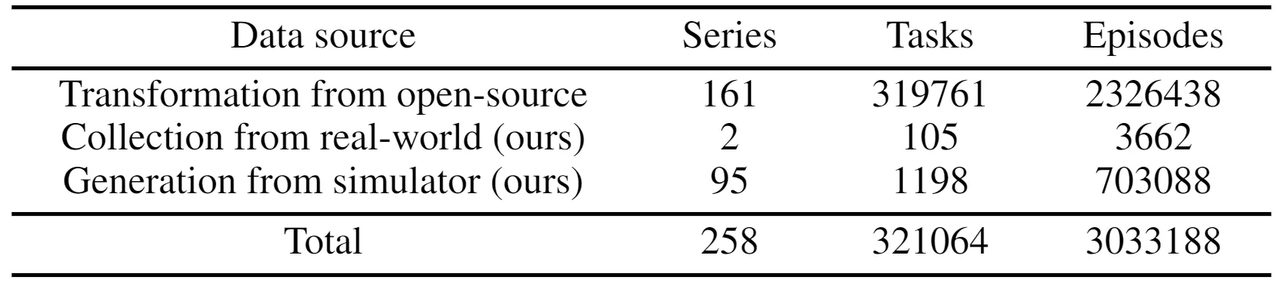
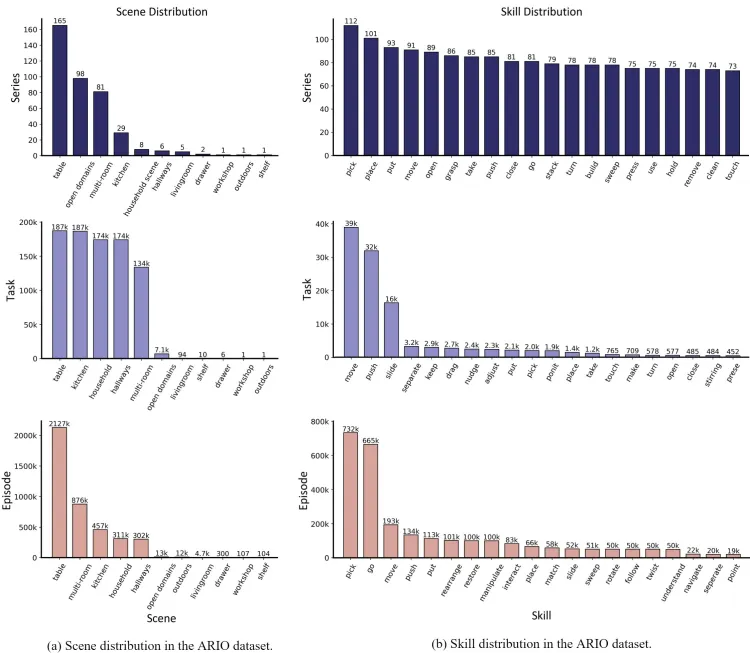
Ario Dataset Scenarios and Skills
- Data Collection: Based on the AgileX Cobot Magic master-slave dual-arm robot platform, scenes and tasks were set up in real-world environments for human data collection. Over 30 tasks were designed, categorized into simple, medium, and difficult levels of operation. Diversity was increased by adding distracting objects, randomly changing object and robot positions, and altering the setup environment. Ultimately, over 3,000 trajectories containing data from three RGB-D cameras were collected.
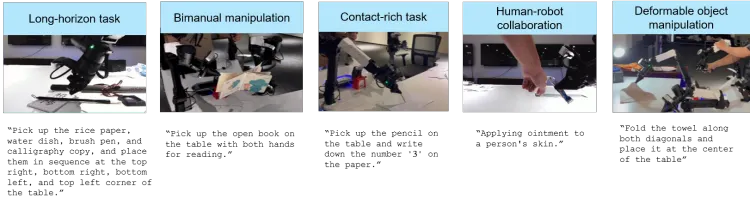
Ario Skill Task
10. RoboMIND
- Publisher: National-Local Joint Humanoid Robot Innovation Center, Peking University, Beijing Academy of Artificial Intelligence, etc.
- Release Date: December 2024
- Project Link: https://x-humanoid-robomind.github.io/
- Paper Link: https://arxiv.org/abs/2412.13877
- Dataset Link: https://zitd5je6f7j.feishu.cn/share/base/form/shrcnOF6Ww4BuRWWtxljfs0aQqh
- Description: RoboMIND is collected through human teleoperation and includes comprehensive robot-related information, such as multi-view RGB-D images, proprioceptive robot state information, end-effector details, and language task descriptions. Researchers not only released 55,000 successful transportation trajectories but also recorded 5,000 real-world failure case trajectories. Robot models can explore the causes of failures by learning from these failure trajectories, thereby improving their performance through this learning experience. This technique represents Reinforcement Learning from Human Feedback (RLHF), where human supervision and feedback guide the model's learning process to produce more satisfactory and accurate results.
- Scale: The RoboMIND dataset contains data from four different robot entities, totaling 55,000 trajectories across 279 tasks, 61 different object categories, and 36 manipulation skills.
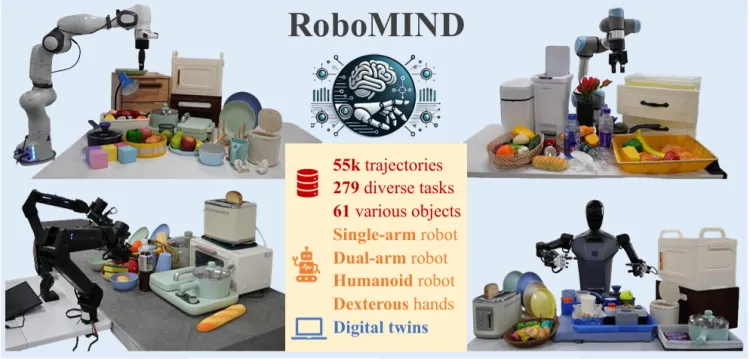
RoboMIND: Benchmark on Multi-embodiment Intelligence Normative Data for Robot Manipulation
- Scenarios: RoboMIND includes over 60 object types from five usage scenarios, covering most daily life environments: home, industrial, kitchen, office, and retail.
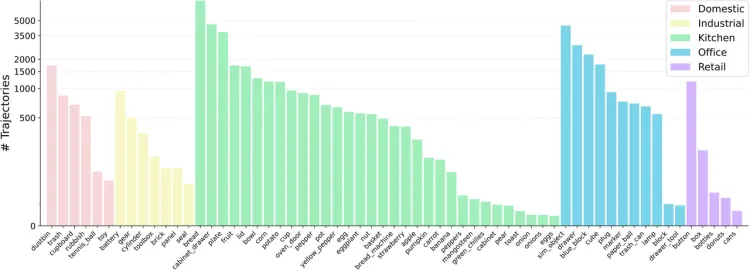
Five Major Scenes of Robomind
- Skills: All tasks are divided into six types:
- Articulated Manipulation (Artic. M.): Involves opening, closing, turning on, or turning off objects with joints.
- Coordinated Manipulation (Coord. M.): Requires coordination between the robot's dual arms.
- Basic Manipulation (Basic M.): Includes basic skills such as grasping, holding, and placing.
- Object Interaction (Obj. Int.): Involves interactions with multiple objects, such as pushing one cube over another.
- Precision Manipulation (Precision M.): Necessary when objects are difficult to grasp or target areas are limited, such as pouring liquid into a cup or inserting a battery.
- Scene Understanding (Scene U.): Relates to the main challenges of understanding scenes, such as closing the upper drawer from the right side or placing four differently colored blocks into correspondingly colored boxes.
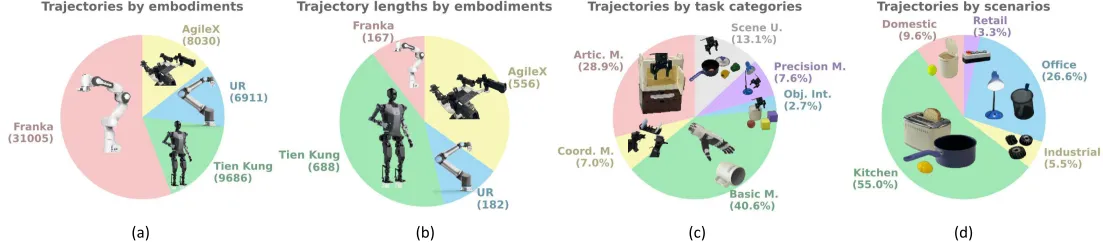
Robomind Task Distribution
- Data Collection: RoboMIND integrates data from different robot types, including 19,222 motion trajectories from the Franka Emika Panda single-arm robot, 9,686 motion trajectories from the Tien Kung humanoid robot, 8,030 motion trajectories from the AgileX Cobot Magic V2.0 dual-arm robot, 6,911 motion trajectories from the UR-5e single-arm robot, and 11,783 motion trajectories from simulations.

Robomind Data Collection
11. RH20T
- Publisher: Shanghai Jiao Tong University
- Release Date: July 2023
- Project Link: https://rh20t.github.io/
- Paper Link: https://arxiv.org/abs/2307.00595
- Dataset Link: https://rh20t.github.io/#download
- Description: Researchers aim to unlock the potential of agents to generalize hundreds of real-world skills through multimodal perception. To achieve this, the RH20T project collected a dataset containing over 110,000 contact-rich robot manipulation sequences. These sequences cover a variety of skills, scenarios, robots, and camera viewpoints from the real world. Each sequence in the dataset includes visual, force, audio, and motion information, as well as corresponding human demonstration videos.
- Scale: The RH20T dataset encompasses diverse skills, environments, robots, and camera viewpoints, with each task containing millions of <human demonstration, robot operation> pairs and a total data volume exceeding 40TB. Each sequence in the dataset includes visual, force, audio, and motion information, as well as corresponding human demonstration videos.
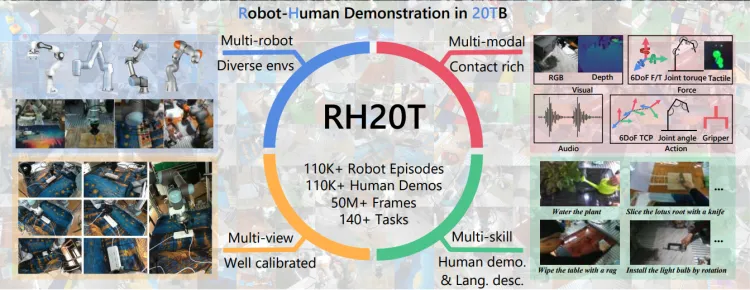
RH20T: A Comprehensive Robotic Dataset for Learning Diverse Skills in One-Shot
- Skills: Researchers selected 48 tasks from RLBench, 29 tasks from MetaWorld, and introduced 70 self-proposed tasks that robots frequently encounter and can accomplish.
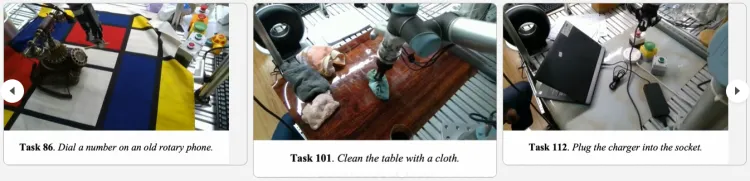
- Data Collection: Unlike previous methods that used 3D mice, VR remote controls, or simplified teleoperation interfaces, this project emphasizes the importance of intuitive and accurate teleoperation in collecting contact-rich robot manipulation data. Each platform includes a robotic arm with torque sensors, a gripper, 1-2 handheld cameras, 8-10 global RGB-D cameras, and 2 microphones for data collection. Using haptic devices and pedals, operators can intuitively teleoperate the robots. All devices are connected to a data collection workstation.
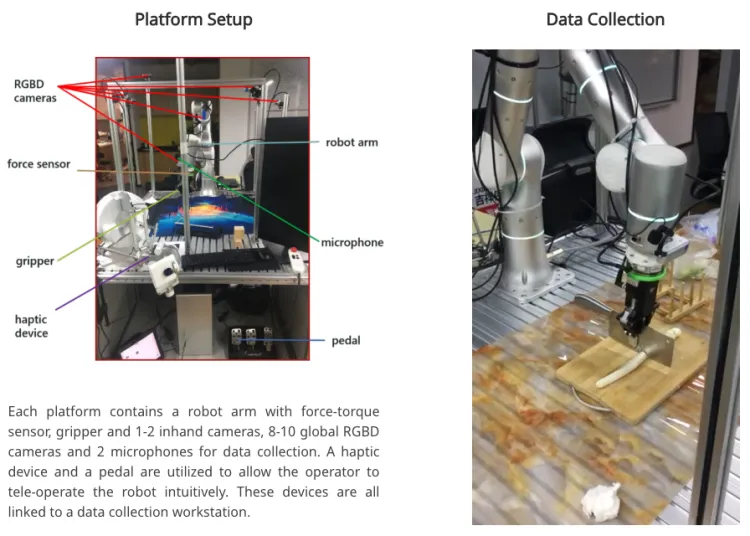
RH20T Data Collection